Outlook on the XMANAI industrial demonstration cases
The key goal of XMANAI project is the Explainable Artificial Intelligence, a type of AI that aims to address how black box decisions of AI systems are made, inspecting and attempting to understand the steps and models involved in decision making to increase human trust. The chance to explain the prescriptions that an AI application may provide to business stakeholders’ decisions could be quite important for the establishment of the solution itself by human beings.
XMANAI will be tested and validated in real-life settings in 4 carefully selected demonstrators involving manufacturing value chain stakeholders, diverse actor’s needs and data sources, heterogeneous industrial systems/ assets, and spanning heterogeneous Industry 4.0 challenges, to showcase the value generated out of the XMANAI AI platform.
Ford Demonstrator
Up until today, it is still very common for factories to suffer unplanned stops in any of their shifts, which means not being able to carry out work in an optimal way due to availability issues. Many times, these unplanned stops are due to defective components, worn out or unwanted conditions of the machine that affect its operation, such as dirt.
The mentioned causes do nothing but reduce production performance in addition to increasing production costs. For this reason, the participation of Ford in XMANAI project is focused on the application of artificial intelligence techniques on corporate production reporting systems, batches, quality, life tools, maintenance actions, etc. with the aim of implementing a system of recommendations that help to optimize and maximize production.
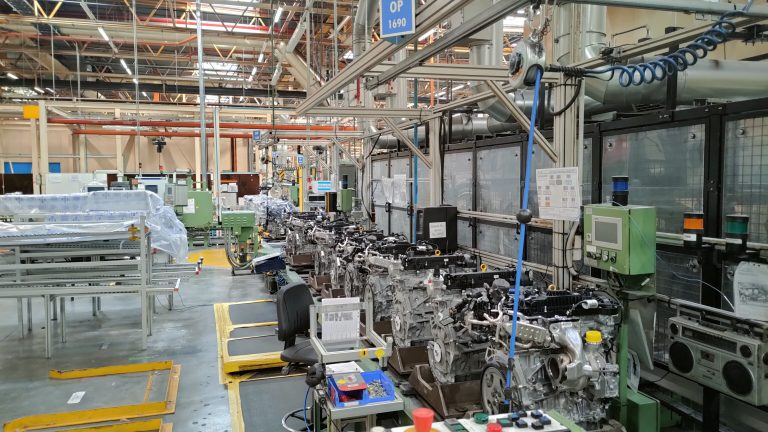
With the help of the XMANAI AI platform, Ford will build novel AI models that contribute to the provision of recommendations to optimize the line throughput of the current and successive shifts.
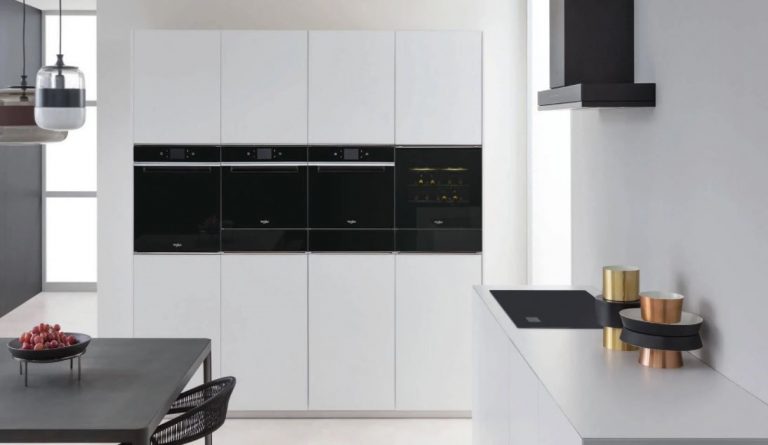
Whirlpool Demonstrator
Whirlpool demonstrator is expected to support the knowledge acquisition of the dynamic related to one of the most complex business processes: the sales demand forecasting.
In particular, the business use case will focus on the Direct To Consumer demand forecasting, business channel that has been only recently launched and activated in Whirlpool EMEA markets, being then constrained by a low level of awareness, knowledge and expertise of business stakeholders.
The trial implementation will lead to the creation of an AI platform capable, not only to generate effective and reliable input for demand management decision taking but also to create the visibility on the relationship among the key factors, creating a real awareness and ownership of the process dynamic.
The key XMANAI features will make possible the production a reliable demand forecasting (AI) and the capability to ensure the visibility of the dynamics and correlations, increasing the capability to understand complex multi-parameter processes and to take better decision based on acquired knowledge.
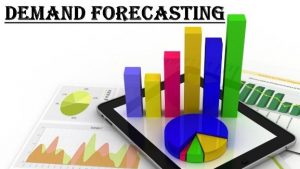
CNH Industrial
A recurring problem suffered by factories today is the unplanned stops, it is an especially important issue in the case of companies that have manufacturing centers and assembly centers.
The stops of the manufacturing centers can be of various causes such as, for example, that the manufactured parts are not of the desired quality and are not suitable for assembly, failure of the line, malfunction due to bad conditions etc.
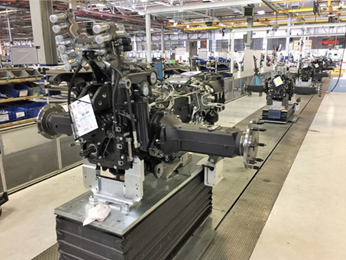
The goal of the CNHi demonstrator is to create a Manufacturing App capable of contributing to the provision of recommendations to optimize the process, product quality, and line throughout the current and successive shifts. Thanks to XMANAI project CNHi will create a more organized data management, sharing, and generate knowledge graphs with clear relationships between data and defined actions. The hybrid and graph AI models will simulate different scenarios related to optimizations and predictions such as product quality based on line efficiency, product measurements, and the tool change strategy based on line efficiency and tool life.
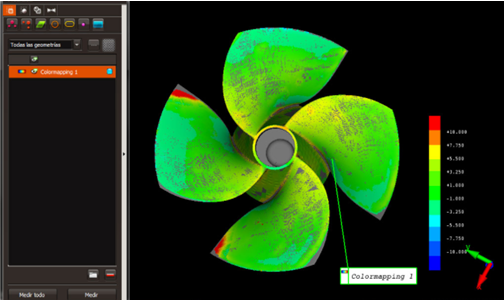
Unimetrik Demonstrator
At present, the technology used for the realization of dimensional quality controls is an optical technology, since it allows the acquisition of a large amount of information in a much shorter time than probing technology. As a consequence, the number of pieces controlled is much higher.
On the other hand, the amount of information that is handled is much greater, a point cloud of a car door can have 15 million points; therefore, the management and calculation algorithms have to be optimized to the maximum.
Through the demonstrator Unimetrik will accelerate the programming and design of quality control strategies during the strategic planning phase, the final objective is to assist metrological engineers and technicians in their preliminary metrological study of the part to be analyzed. The metrological study is a long process and, with the help of AI models, XMANAI will increase the speed of metrological analysis and guide engineers to plan strategy finding new innovative methods. Explanations of why certain decisions/recommendations are made will keep engineers on a dynamic cycle and speed up the analysis in an informed manner.