UNIMETRIK Pilot
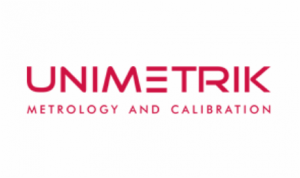
Unimetrik is a Metrological Service company, oriented to offering solutions for the industry related to Calibration, Measurement, and Metrological engineering. UNIMETRIK is accredited by ENAC and acknowledged by the principal aeronautic groups in Spain. It is exporting its technology to countries such as the USA, Germany, Brazil, and the Czech Republic.
Unimetrik is part of Innovalia Metrology, with the strategy of creating and developing projects in a flexible way. For this reason, multi-disciplinary teams are formed, joining efforts from different companies in order to achieve the particular objectives of each project. The team at the company holds excellent relations established with the biggest Technological Centers in the world. All the group meets the technical and administrative requirements, which together with its wide knowledge of this sector in the Basque Country, Spain, and the rest of the world, facilitates the growing penetration in the global market by Unimetrik.
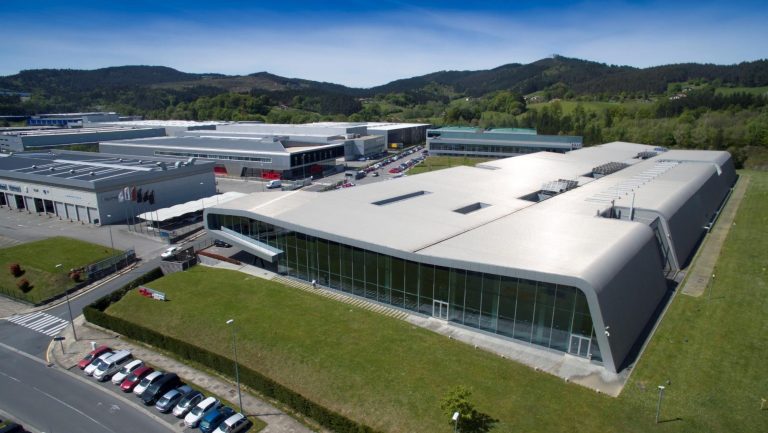
Description of the Objectives
The main goals of UNIMETRIK when participating in the XMANAI project, are:
- To reduce the measurement time.
- Predict, based on certain key parameters, the level of reliability of the result.
- To minimize the effect of errors in the manufacturing process due to deviations in measurement.
In order to fulfill these goals, the company is facing various challenges such as:
- Knowledge transfer from experienced metrologists: Highly experienced metrologists are able to adequately define the measurement strategy in the M3 software (specific measurement software used in metrology). Based on their great experience they know which regions of the piece to be measured are the most relevant, and so, they are able to define accurate point sets. This is a relevant part of the measurement process where the XMANAI platform would help.
- Reuse information from previous measurement procedures: When the metrologists start to define the measurement strategy of a part, none of the previously performed jobs are used. The strategy is defined from scratch for each job. The XMANAI platform will contribute to “reusing” relevant information such as key parameter value configuration.
- Understand the impact of different scanning configurations: Setting different values for the scanning main parameters (such as Point Cloud lateral density, scanning direction, and exposure time) leads to measurements with different quality and accuracy. It is a challenge not only to identify the correct values for the scanning parameters but to understand why and how they impact the accuracy of the measurement.
- Extrapolation of data from scratch: Designing a measurement plan is very time consuming because the data that the program needs to proceed with the measurement is entered by hand by each metrologist. The Unimetrik demonstrator faces the challenge of being able to extrapolate data from previous jobs in case of reusing values from another measurement, the data to program the machine is entered by hand, which supposes a considerable loss of time.
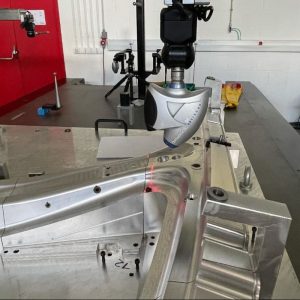
Use Case
The UNIMETRIK pilot is divided in two different use cases that are related to each other.
Use case 1: Measurement plan optimization
- Assist the junior metrologists in their preliminary study of the part to be measured
- Accelerate the process & minimize costs
- Ensure consistency of results
Use case 2: Point Cloud optimization
- Maximize measurement accuracy
- Minimize execution time
- Ensure consistency of results
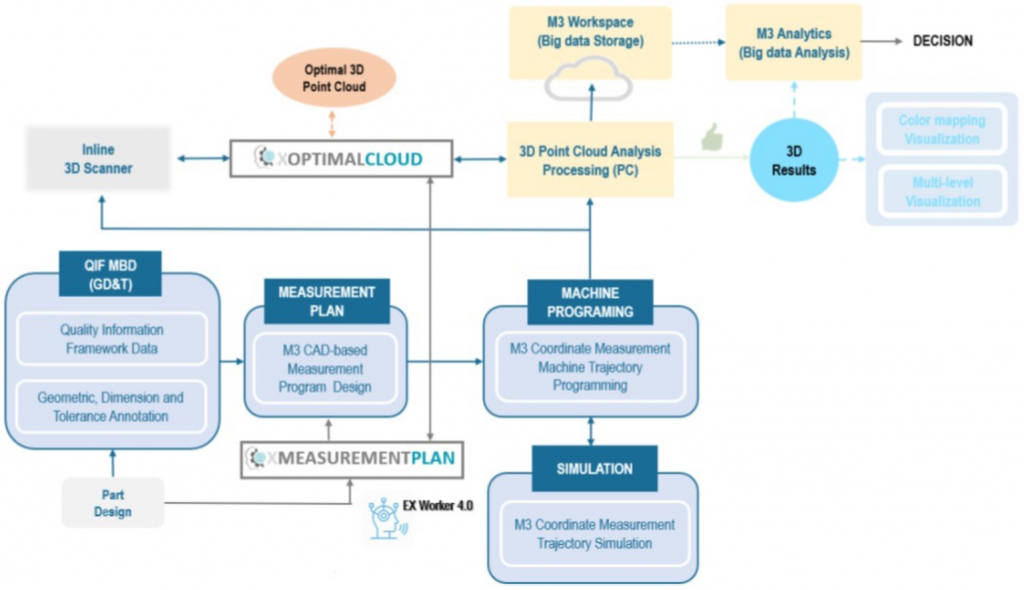
To solve these challenges regarding the both use cases, the first datasets for experimentation have been generated, as dimensional measurements of calibrated objects with geometries that are common to industrial metrology studies, such as the sphere and the cylinder. Starting with measurements of calibrated spheres of three different diameters, and moving on to a calibrated cylinder, the focus is on convex geometries to begin with, due to the different response of the scanning instrument to concave geometries. Exploratory data analysis has been performed on the available datasets, uncovering interesting properties, interrelations and hidden patterns in the data. Informative features have been extracted from the available data, taking into account the laser setup and the geometric characteristics of the object under study. These geometrical features are used as inputs to the ML models, along with the combination of scanning parameters that has been applied to each measurement.
The raw point clouds obtained by measuring objects with those 3 different geometries have been processed to achieve Outlier removal (by differentiating inlier and outlier points), estimation of Surface Orientation vectors (at each point to ensure they are aligned) and Geometric feature extraction.
Regarding the development and validation of Explainable Artificial intelligence models and algorithms, several machine learning algorithms have been applied to the problem, and moreover, selected models have been trained and fine tuned as SVM + Permutation Importance and NN + SHAP.
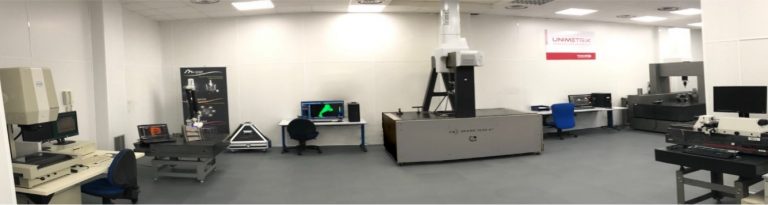
Explainability Expectations and Take-up
The XMANAI solutions should help UNIMETRIK with:
- Setting a Measurement plan:
The metrologist (no matter if it is an experienced one or a junior metrologist) needs to set the best scanning configuration, so that the smallest measurement error is provided as a result. To do so, the interface of the XMANAI platform will gather input from the operator (the metrologist) on the desired measurement error and will formulate the congruent scanning configuration.
- Visualise Explanation:
The metrologist is in need of visual feedback on the results of the effect/impact of different scanning configurations over the precision of the measurements. By getting this visual information, the metrologist will be able to set the parameters according to the explanation and the impact of different methods on the final measurement. To do so, the interface of the XMANAI platform will produce a visual representation of the impact of the different parameters (for example a heatmap), SHAP plots explanations, or even decision tree and textual explanations as the metrologist may not be able to understand SHAP plots or similar kind of graphs.
- Perform Quality Check:
The metrologist must be able to validate the accuracy of the suggestions given by the model against the real values to that the suggestions can be tested to understand how good or bad the model is. To do so, the XMANAI platform will let know to the metrologist the correlation matrix of different measurement parameters against the initial scanning settings.
Explainability studies have been performed for the UNIMETRIK demonstrator aiming to cover the needs of the business user in understanding the predictions of the AI models, but also the characteristics of the data used to train these models.
Data Description
In terms of Data, for the UNIMETRIK pilot the Dataset is made up of Point Clouds as a result of measurements of different calibrated objects with common geometries as Spheres or Cylinders, and QIFs, as a standard for the use of metrology-related information.
With each measurement, the data file formats provided are .CSV , .QIF and .TXT with an aprox size of 50 Mb. But it is important to point out that the size of the point clouds depends on the complexity of the geometry and measured part.
It is also important that the Dataset used by the XMANAI platform for the UNIMETRIK demonstrator does not use updates, as the whole Dataset will change for any new measurement project as a result of the metrology process and the M3software itself.
XMANAI platform components
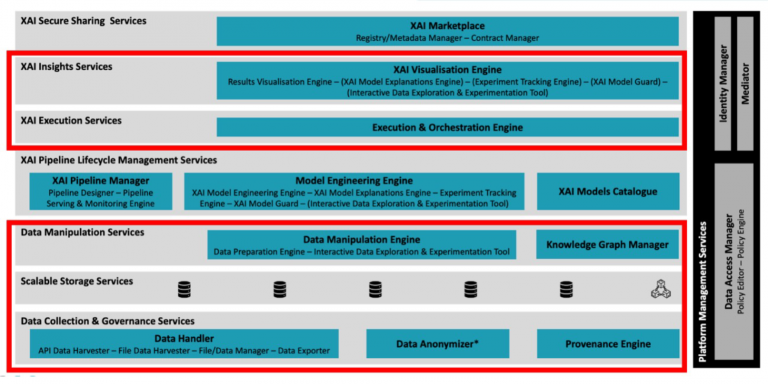
The UNIMETRIK Pilot will use several components of the XMANAI platform, but due to the own special characteristics of the metrological sector, certain components such as de Data Manipulation Engine or the XAI Data Model will need additional efforts to fulfill the pilot goals regarding the visualization Dashboard.
In terms of Models and Algorithms related to the UNIMETRIK demonstrator, the first problem to be solved is formulated as a supervised regression task. The algorithms selected to address this task are the Support Vector Machine (SVM) and the fully connected, artificial Neural Network (NN) regressors. These models are highlighted in preliminary experimental results on sample data, due to their satisfactory performance and their continuous decision functions.
Complementary to the employed ML algorithms, a couple of well-established explainability techniques have been selected to explain their predictions. In particular, Permutation Importance and SHAP values constitute the explainability components that are coupled to the SVM and NN regressors, for the construction of the associated Hybrid XAI models. Both techniques provide post-hoc feature attributions for the trained models, explaining which features have the greater effect on the model’s outputs. Permutation Importance provides global feature attributions, to explain the overall behaviour of the trained SVM. SHAP values on the other hand are calculated on the local scale (i.e. for each feature and each training sample) and expose more information on the trained model’s underlying mechanics, explaining which features and how they affect the trained NN’s individual predictions. Adding to that, explanations at the global scale are deduced by aggregating the computed local feature attributions.
Manufacturing apps being developed
The development of the manufacturing app for the UNIMETRIK pilot is under development but, taking into account the previous requirements regarding both the business and the explainability areas, the following mockups are the starting point for the successful development of the application.
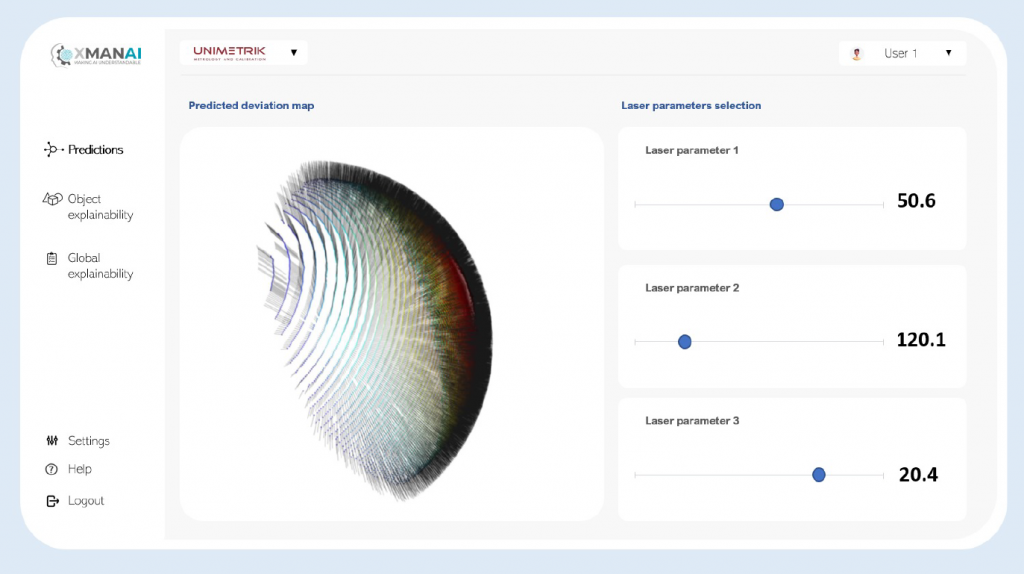
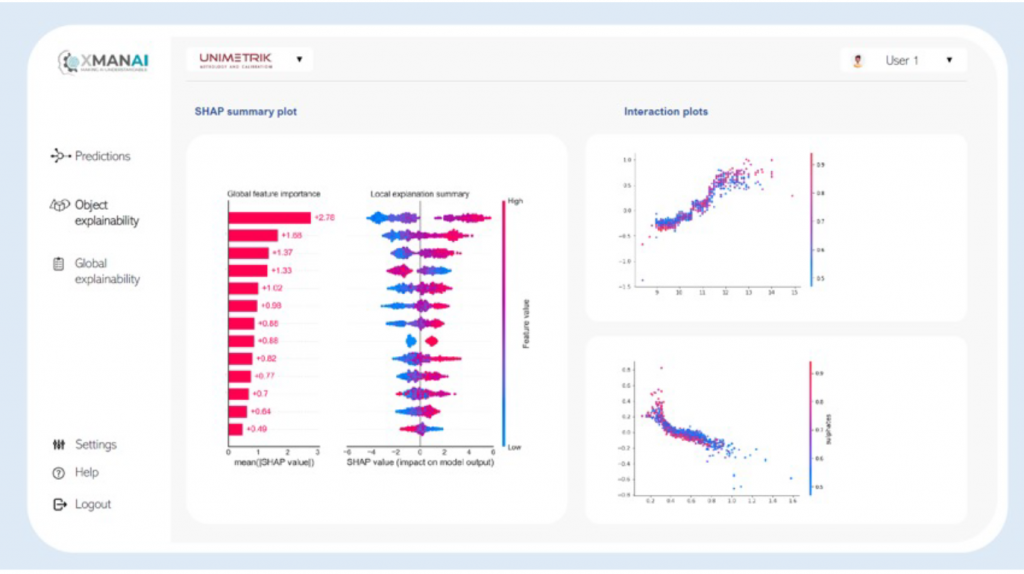
Results
The stage of Data acquisition & analysis, feature extraction and the Development & validation of Hybrid XAI models are being and will be for the next version of the platform, repeated to include new measurements that are relevant to the first use case and are made available for experimentation.
The inclusion of additional geometries to the already existing dataset will allow for the models to capture the overall response of the scanning instrument to convex/non hull objects, so that this family of solutions is finalized and we can proceed with the analysis of concave objects. Given enough measurement data that cover diverse geometries with a fine granularity of scanning parameter values, a complete solution is expected to be reached under the established approach. The final models shall have the ability to generalize to unseen objects.
Having formulated a concrete approach to the prediction of the scanning instrument’s differential response, the UNIMETRIK pilot team is examining possible ways to relate predicted point measurement deviations to the final result of the measurement (dimensional measurement and tolerance). This interrelation will lead to predicting the optimal scanning parameter values for a given measurement, solving Use Case 2.