Whirlpool Use Case
Whirlpool Corporation (NYSE: WHR) is the world’s leading kitchen and laundry appliance company, with approximately $19 billion in annual sales, 78,000 employees and 57 manufacturing and technology research centers in 2020.
The company markets Whirlpool, KitchenAid, Maytag, Consul, Brastemp, Amana, Bauknecht, Jenn-Air, Indesit, Hotpoint and other major brand names in nearly every country throughout the world.
In Europe, Middle East and Africa (EMEA) it has approximately 19,000 employees, a sales presence in more than 35 countries and manufacturing sites in 5 countries. Whirlpool EMEA is an operating segment of Whirlpool Corporation and Whirlpool Corp.’s EMEA Headquarters are located in Pero (MI), Italy, where most of central functions are hosted.
In Italy, besides the managerial centre, Whirlpool owns its Centre of Excellence for Research in Cassinetta, (Varese district), and in Fabriano (Ancona district), employing researchers and technicians working on the development of innovations which are transferred to all EMEA businesses of the group. The Research Division has acquired over the years expertise in numerous areas such as manufacturing, materials, mechanical design, thermodynamics, energy transmission, energy efficiency, etc., that enable the company to undertake significant and challenging research projects. Strategic collaborations with several universities and research centres from all around EMEA support Whirlpool needs.
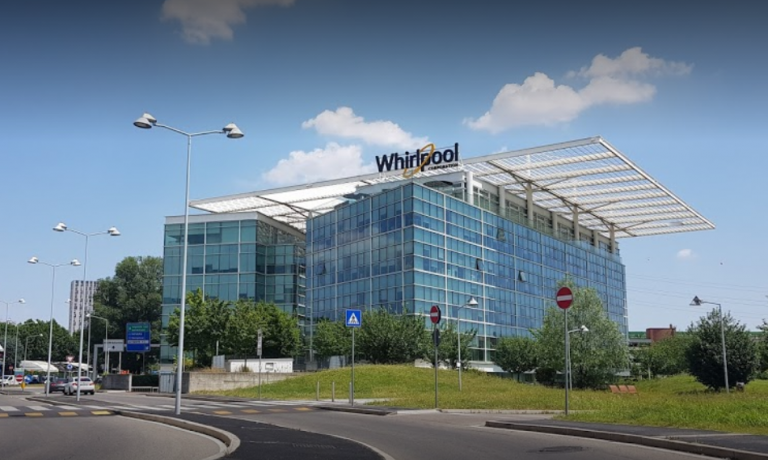
Description of the Objectives
The Whirlpool use case in the XMANAI project has the main objective of creating robust support for the decision-making process for Direct To Consumer (D2C) market business management. The basic element is represented by the generation of reliable demand forecasting for the D2C market, creating clear evidence of the expected sales per product and date in order to properly set up the supply chain process ensuring the required product availability. The AI will then support the central demand planning team to make the best decisions in order to provide the right product, in the right quantity, at the right moment to be offered to the customer’s buying experience, maximizing customer satisfaction and minimizing the product inventory value. The same information will also support the D2C marketing and sales team to properly execute the right market actions (pricing, promotions, product range offered, …) to maximize the margins and boost the profit.
Definitively, this objective is neither the most important as the XMANAI platform is expected to offer the users the possibility to get explanations about the generated forecast, further supporting the decision-making process and also deeply understanding the dynamics of the business behavior. The key enabler of AI adoption in the mid-long term is, without any doubt, the “trust” that users have to achieve to proficiently interact with the system and this has mandatory to pass through the XAI as the capability of the AI system to explain the reasons of a specific result. The XAI functionality will then allow the users to understand the correlation among the different factors, generating the forecast result and directly experimenting with the effect of each of them on the final outcome. This will enable a deep understanding of the factors that may be driven to achieve the expected sales results, leading to higher business performance and customer satisfaction.
Use Case
Whirlpool's use case is focused on 2 groups of stakeholders:
1. The business roles: Central demand planning, D2C marketing, and sales: they’re interested in getting access to the forecast result and in the explanation of the reason why it has been generated with specific values. They’ll have a dashboard for the demand profile visualization and for the visualization of the key features which have generated it. They’ll have visibility about the relations and correlations among the different features and they’ll have the possibility to simulate the effect of specific values of the forecasting process and, on the contrary, have evidence of the combination of values to generate a specific expected forecast (“what if” simulation). They’ll have also the possibility to get evidence of anomalies, trends, patterns, behavioral schemas, and the features which have generated them.
2. The technical roles: data engineers and data scientists: they’re interested in maintaining AI and XAI functionalities performances, and managing data pipelines and algorithms, in order to ensure minimal forecasting error. The Demo sessions will be held in 3 phases: the first involves Central demand planning for the preliminary AI validation of demand forecasting and for XAI functionality on demand profile and what-if simulation. The feedback will be captured for system improvement and for the preparation of the second phase. This will involve also D2C marketing and sales with a special focus on XAI functionalities: Also, in this case, the feedback will be captured for the final demo session. This third one will be held fully replicating daily operations and the platform will be used for a period of 2 months to properly capture the measures of project KPIs. For all the phases the technical roles will be involved as validators and system administrators.
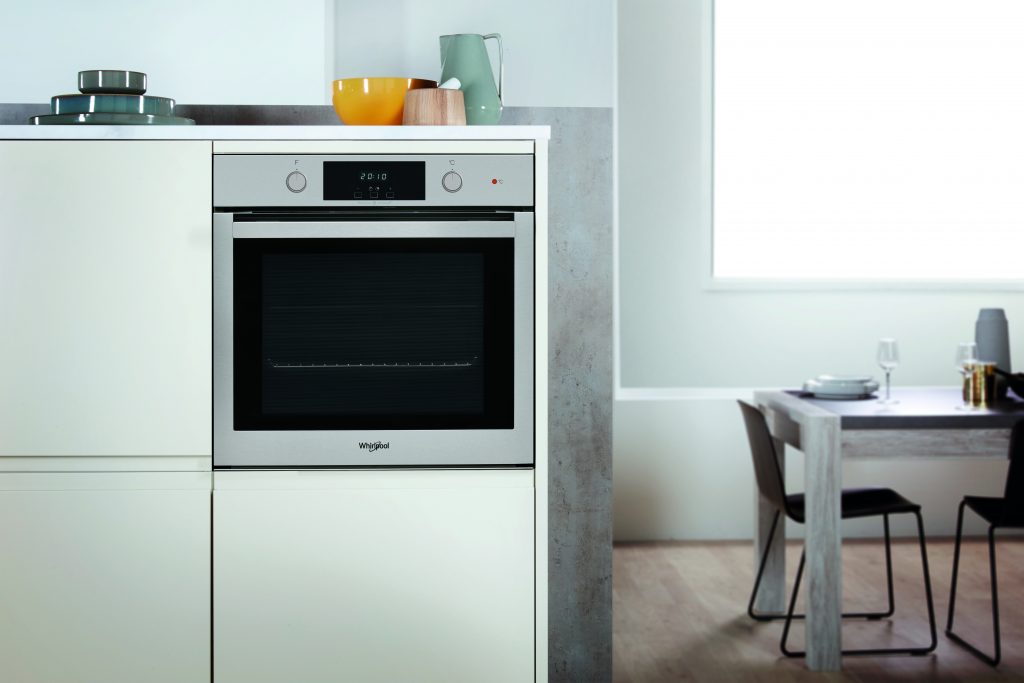
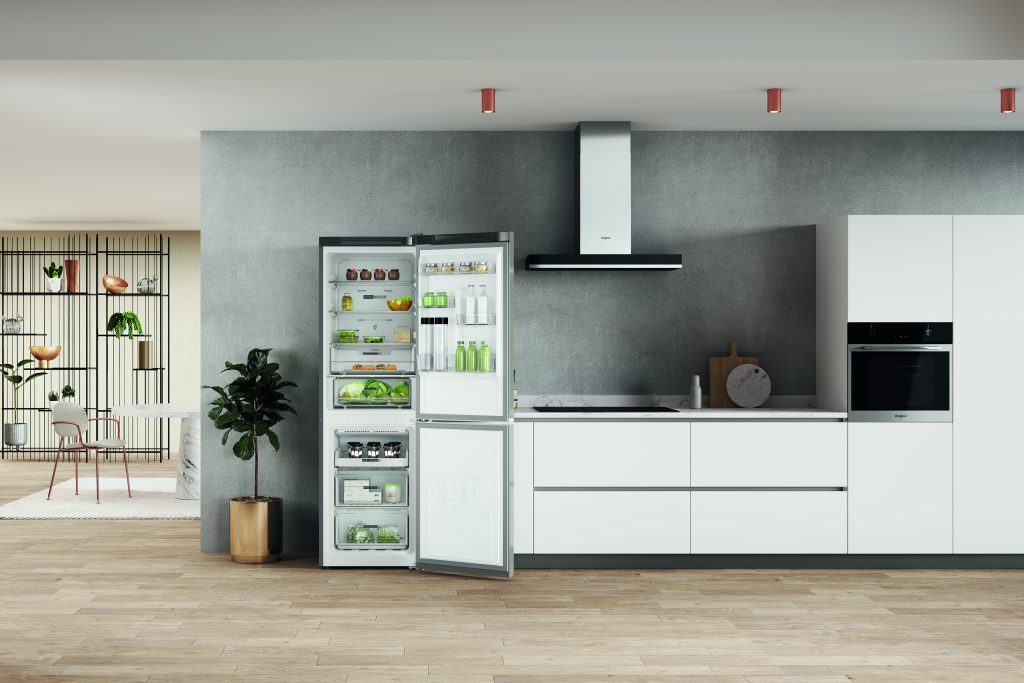
Explainability Expectations and Take-up
The XAI expectation of the business roles is to be able to use the XMANAI platform as a valid support to the daily decision taken in the D2C business management. The central demand planning team is expected to use Explainability to correctly understand the demand evolution and arrange supply chain capacity to match customers’ demand.
The D2C marketing and sales are expected to get out of the Explainability function the knowledge about the customers’ desires and expectations in order to set up sales actions capable to match their desires and stimulate their buying experience.
Data Description
The data that the XMANAI platform will ingest for Whirlpool’s use case is mainly referred to D2C sales and google analytics related to the Whirlpool D2C website usage by the customers. These data are integrated and completed by product master data and promotions data to ensure a reliable forecasting process.
XMANAI Platform Components
XMANAI platform will be deployed in phases, similar to the user’s demo calendar, in order to support iteration for system improvement. The first interaction, to support the first demo phase, will be deployed with the following components.
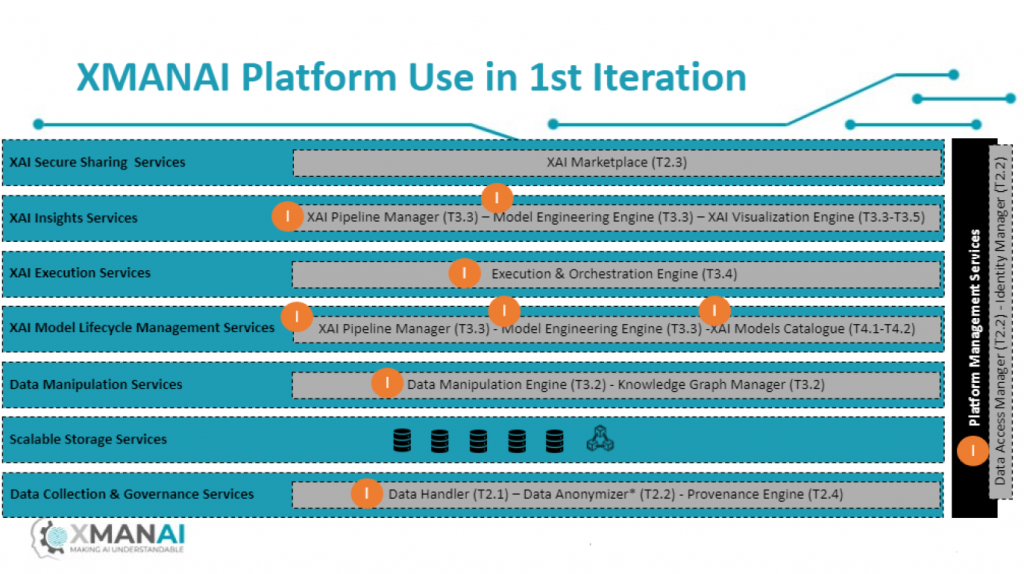
Examples of visualizations
The XAI functionality will be deployed to the users through a dashboard that will visualize the various elements capable to create a deep understanding of the reason for a specific forecasted value in the demand profile.
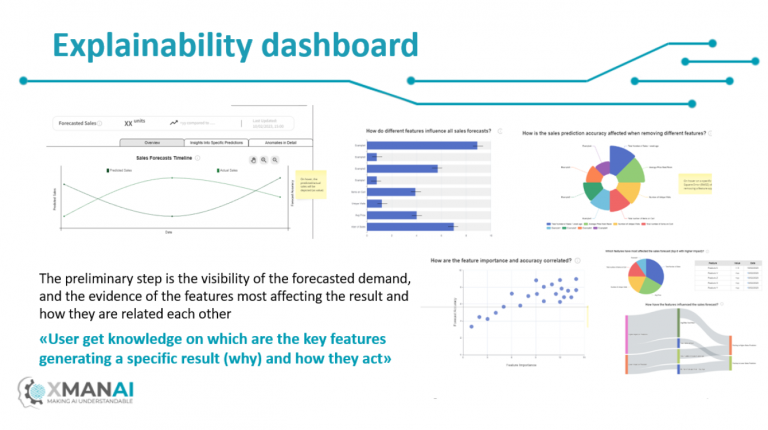
Also the anomalies and their root cause might be visualized through the dashboard:
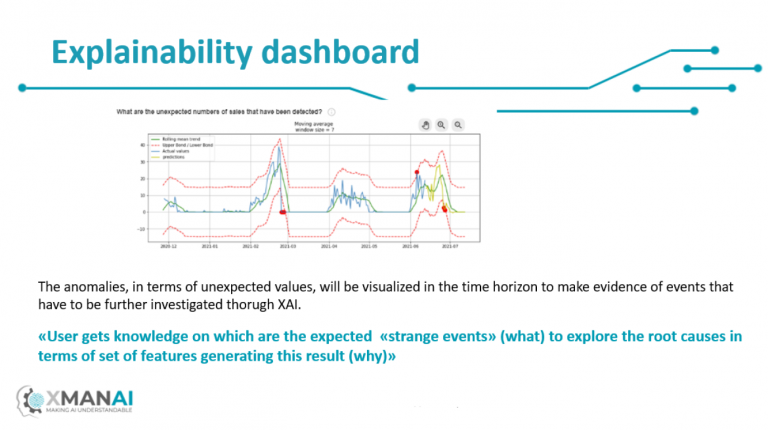
Like for the buying patterns of customer behaviors schemas:
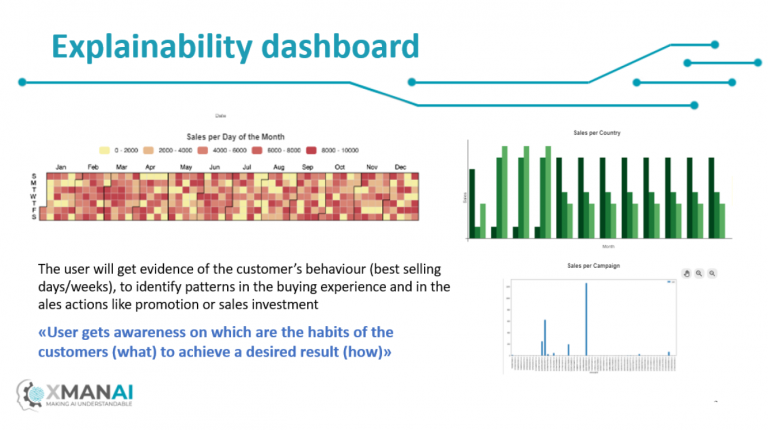
All this information will help users deeply understand the business process dynamics in order to allow them to control it for the best performance achievement.
Results
Key benefits are expected by the successful completion of the demonstrator both in terms of business results and of people knowledge maturation:
- Customer satisfaction
- Revenue’s opportunity maximization
- Tailored inventory strategy
- Promotional actions effectiveness
- Product range optimization
- Business channel order to deliver process optimization
The project success will be measure through specific KPIs, focused on the measurement of the effectiveness of the introduction of the XAI solution supporting business channel management:
- DFE (Demand Forecast Error) monthly and weekly, measuring the reliability of the sales forecast generated by AI: <25-30% and <50% respectively
- ATP (Availability To Promise), measuring the organization’s capability to understand business dynamics ensuring the right products at the right moment for the customers: 98%
- Revenue increase, measuring the organization’s capability to understand business dynamics key factors maximizing sales opportunities: +10% vs. Other similar channels without XAI technology support.
- 5STARS, measuring customer satisfaction and company reputation improvement driven by a higher service level of the business channel: 4-5.