Holistic Production Overview using XAI technologies: The FORD use cases
Ford Motor Company is a global automotive and mobility company. The Company’s business includes designing, manufacturing, marketing, and servicing a full line of Ford cars, trucks, and sport utility vehicles. Ford plants and offices are located in every region of the world, employing in 2020, around 186,000 people. In total, there are 6.883 employees and we produce 267k vehicles per year. The pilot of the project focuses on the engine plant located in Valencia, Spain.
The core issue lies in managing the complexity and variability of manufacturing at the Valencia engine plant. With 25 different derivatives in production, each requiring various components, the current manual system of weekly batch planning by engineers and decisions made by shift foremen creates bottlenecks, impacting plant performance.
Holistic overview of the production use case
Holistic overview of the production with real-time representation of the production line, unwanted scenarios alert system and workload simulations. This use case is divided in three different tasks:
• Real-time representation of the production lines, visualizing the status of each of the operations, whether they are automatic operations, manual stations, warehouses and so on. From this representation, a prediction of the number of the total engines will be produced within a production shift. If there are deviations from the expected number of engines and the predicted number, it will be important to identify which elements of the line are contributing to this deviation. This information will be useful for the users to infer the root causes of the deviation and to provide a quick response.
• A system for alerting of unwanted situations that may lead to loss of line efficiency, based on real-time information provided by different data sources. Similarly, to the first point, the explainability needs here are the same.
• Simulation of process changes. This task focuses on determining what would happen if some of the parameters of the production process were changed, using the data learned from the operation of the production line, machine states, efficiencies, cycle times per model, etc. For example, if the cycle time of a station for a particular model is increased, how this would affect the overall view of the line.
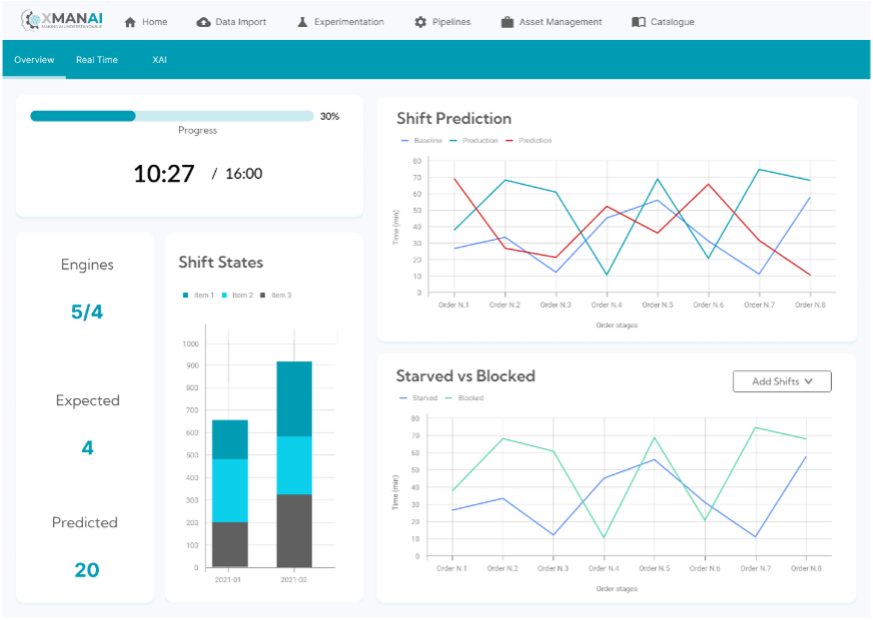
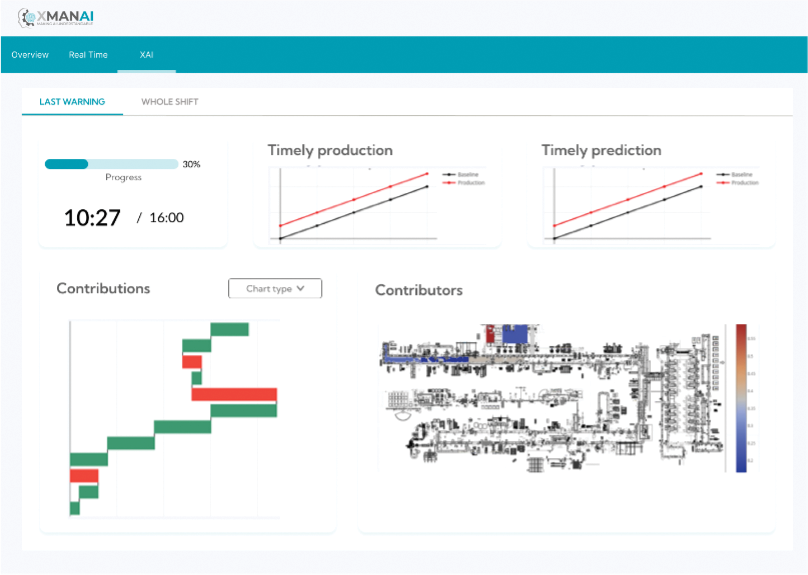
Automated Production Planning use case
This use case consists of developing an intelligent planner that considers the production plan, customer plant demand, available components, current production on the different lines, and so on. With all this information, the planner will recommend which batches should be made on which lines. The explainability comes here through the comparison of different plans and understanding why one is better than the others considering a set of reference metrics.
The Material Planning & Logistic Engineer needs to know “why” it is the best plan of production as this is very important for the plant. This is the key, so we need explainability; conventional artificial intelligence is of no use to us.
We need to update the constraints on production data of the last shifts as the line is constantly changing and fixed constraints do not reflect the current situation. Moreover, we need to check the availability of parts and the plan of production to create the best mix of production based on the current constraints.
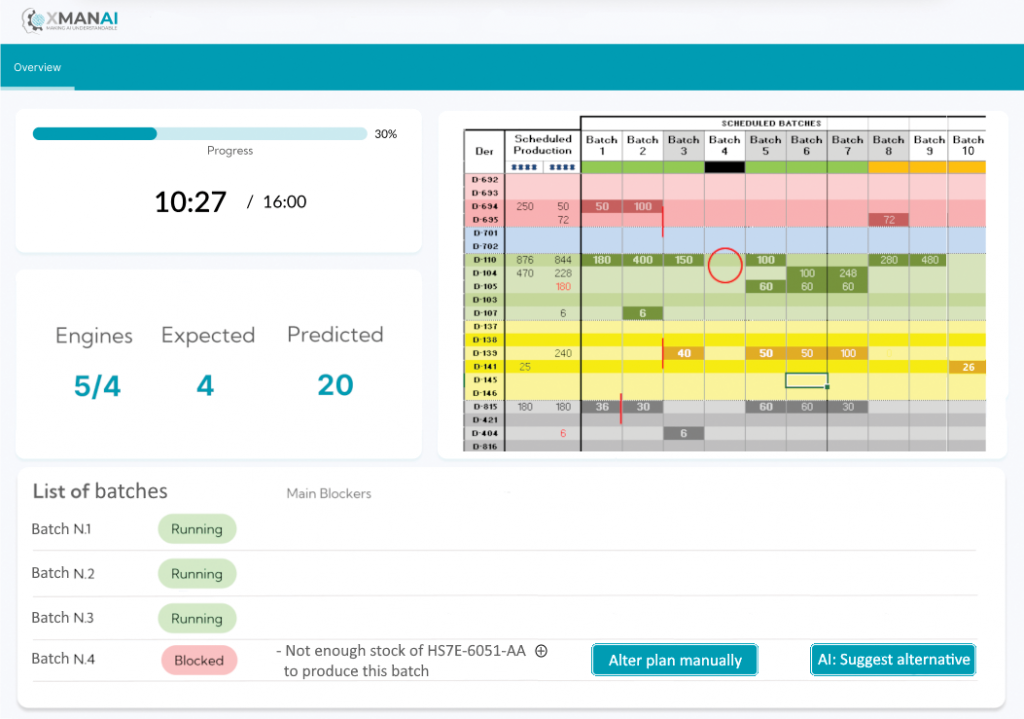
Results
The utilization of XMANAI at Ford not only allows for the integration of cutting-edge algorithms in data analysis through XAI but also brings about tangible benefits for the plant in terms of two key performance indicators:
Firstly, it enhances Availability by significantly reducing downtimes for the manufacturing machines in the implementation of the first use case. This improvement directly contributes to maximizing operational efficiency and ensuring a smoother workflow.
Secondly, it elevates Line Efficiency by optimizing scheduling to achieve the best mix and increase overall production output. By reducing over cycles during the second use case implementation, the plant can operate at peak performance levels, thereby enhancing productivity and minimizing resource wastage.