Industrialization Approaches to Explainability and the XMANAI Cases
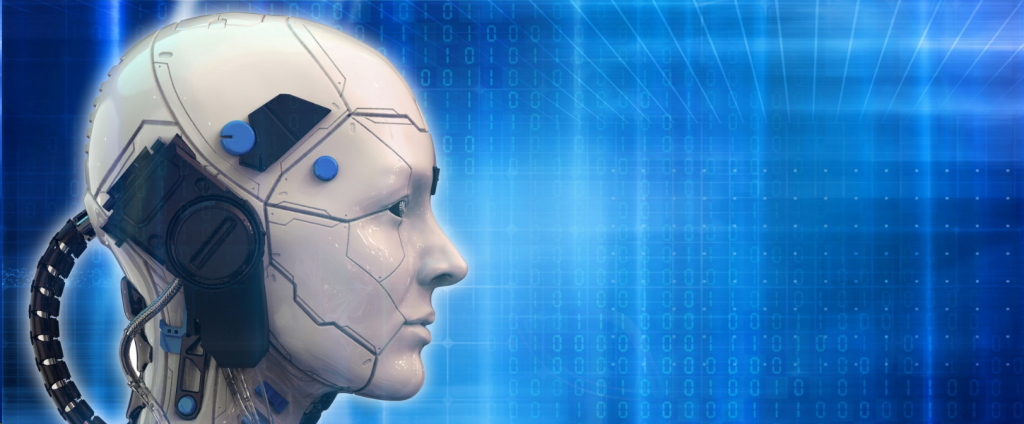
Artificial intelligence (AI) has become integral to modern manufacturing processes, enabling increased efficiency, productivity, and automation. However, most of the current AI systems make it difficult to understand their decision-making processes, leading to concerns regarding transparency and trust. Explainability in AI systems has emerged as a crucial research area, aiming to provide new insights and knowledge on how AI models arrive at their conclusions. This article explores industrialization approaches to explainability, sheds light on the importance of transparent AI systems within the manufacturing sector, and shares the XMANAI cases approach.
Beyond Results Visualization: Explainability
As AI systems become more advanced, their decision-making processes become increasingly opaque, making it difficult to comprehend the principles behind their outputs.
The European Parliament implemented the General Data Protection Regulation (GDPR) as a law in May 2018. One notable feature of the GDPR was its inclusion of provisions on automated decision-making and profiling. These provisions mark a significant development by introducing, to some extent, the right of individuals to receive “meaningful explanations of the logic involved” when automated decisions are made. Although legal scholars hold differing views on the precise extent of these provisions, there is a widespread consensus on the urgent need to implement such a principle and the substantial scientific challenge it presents.
When looking at the manufacturing sector, where AI-powered technologies are employed extensively, explainability is critical for a variety of reasons:
- Enhancing Trust: Transparent AI systems instil trust among blue collar workers and general end users of the AI systems. Knowing how AI models arrive at their decisions fosters confidence in their reliability and ensures accountability.
- Compliance with Regulations: Regulations surrounding AI in manufacturing are evolving rapidly, with an emphasis on ethical and responsible AI deployment as well as traceability. Explainability aids compliance by enabling white collar workers to understand and document the decision-making processes of their AI systems.
- Diagnostic Capabilities: Explainable AI provides valuable insights into system performance, aiding in the identification and rectification of potential errors or biases. This diagnostic capability helps manufacturers improve the quality and reliability of their products and processes, and helps the end users of the AI systems to gain knowledge and experience on their fields.
Industrialization Approaches to Explainability
While the benefits of Explainable artificial intelligence for the Manufacturing sector are clear, the path to deliver them is not yet clearly defined.
To address the challenges of explainability in the manufacturing sector, researchers have proposed various industrialization approaches that bridge the gap between academic research and practical implementation. These approaches include:
- Rule Extraction Techniques: Rule extraction methods aim to generate human-interpretable rules from black-box AI models. By extracting rules that mimic the behavior of complex models, manufacturers gain insight into the decision-making process and can explain the reasoning behind AI predictions.
- Model-Agnostic Techniques: Model-agnostic approaches focus on explaining AI models without relying on internal model details. Techniques such as LIME (Local Interpretable Model-Agnostic Explanations) and SHAP (Shapley Additive exPlanations) analyze the relationship between input features and model outputs, providing intuitive explanations for individual predictions.
- Transparent Model Architectures: Researchers are exploring the development of inherently interpretable AI models. These models, such as decision trees or rule-based systems, offer transparency by design, enabling a clear understanding of their decision-making process. However, they may sacrifice some predictive performance compared to more complex models.
- Post-hoc Explanation Methods: Post-hoc explanations involve analyzing an AI system after it has made a prediction. Techniques like attention mechanisms and saliency maps identify the most influential features or regions in the input data, helping manufacturers understand the factors driving the system’s decision.
Explainability and user scenarios. The XMANAI cases
With the technical approaches already revised, manufacturing organizations need to identify their own specific explainability needs for the artificial intelligence (AI) systems they are implementing. These explainability requirements elicitation is essential to ensure transparency, accountability, and trustworthiness and it will be the key to an adequate and effective integration in the main industrial manufacturing processes.
The explainability take-up path by the XMANAI demonstrators has been made up with a specific roadmap, aimed at gathering the explainability requirements aligned with the previously identified business requirements.

The 4 demonstrators involved in the XMANAI project and the Manufacturing Apps they are developing with the support of the Explainable Artificial Intelligence Platform XMANAI are the following ones:
- WHIRLPOOL: AI for Product Demand Planning
- FORD: AI for Production Optimization
- CNH: AI for Process/Product Quality Optimization
- UNIMETRIK: AI for Smart Semi-Autonomous Hybrid Measurement Planning.
For all these organizations, the starting point was the identification of their own explainability requirements in direct correlation with the business requirements previously gathered. Based on them, Explainable user stories were defined per each type of user (Data scientist, Data analyst, and Business user) in order to identify a scenario were the explanations were needed, and the impact that those explanations would have on the processes aided by the XAI system.
After identifying where to implement the explainability, the next step was to define in which way the users of the 4 manufacturing applications were going to receive the explanations. The explainability output and visualization process first performed classification and categorization of explainability visualizations from literature, matching them with the user stories, and then prioritized the visualization type for each organization and pilot.
This explainability path was implemented in accordance with the technical elicitation of hybrid explainable AI models and their training.

Challenges and Future Directions
Explainability plays a vital role in building trust, ensuring compliance, and improving the overall performance of AI systems in the manufacturing sector.
From a technical point of view, while significant progress has been made in industrializing explainability techniques for AI systems in manufacturing, several challenges remain. These challenges include balancing accuracy and interpretability, developing scalable and efficient approaches for the manufacturing sector as a whole, and addressing the balance between model complexity and transparency.
Taking into account the business users’ perspective, their insights and understanding of the operational context, business goals, and specific needs are invaluable in ensuring that the explainable AI systems align with organizational objectives. By involving business users from the early stages of implementation, organizations can gather requirements, expectations, and user feedback to develop AI systems that provide meaningful and relevant explanations.
Moreover and looking ahead, future research directions should focus on developing unified standards and guidelines for explainability in manufacturing AI systems. Collaboration between academia, industry, and regulatory bodies is crucial to establish best practices, promote transparency, and ensure the ethical deployment of AI technologies.