XMANAI partner spotlight – Fraunhofer FOKUS
Q: What is your organization’s role in XMANAI?
A: Fraunhofer FOKUS is the leader of the working package “Asset Management Bundles Methods and System Designs”. In this working package, management and sharing methods are defined and prototypically implemented for the assets. The assets mentioned are industrial data as well as AI models and analyses based on these data.
We have different user roles in mind, such as the data engineers or the business users. This is because they always have a different understanding of data management and accordingly bring diverse requirements to the project.
In addition, Yury Glikman is the scientific coordinator. He supervises the scientific process and output of XMANAI. For this purpose, he makes sure that the research is state of the art, applicable and in line with the project goals.
Q: How does XMANAI relate with your or your team’s background and interests?
A: With our expertise in data platforms and data management, we are very well prepared for XMANAI in data-driven topics such as data harvesting, data transformation, data cleansing, etc. Furthermore, the data within the project comes from industrial manufacturing and production, which can be used for AI explainability with our experience and applications.
We aim to develop a human-centric and trusted approach based on our 30 years of experience in national and international initiatives of data management, analysis and sharing.
Finding, accessing or re-using data are standard data management operations. Therefore, applying these common operations to an “AI glass-box approach” and extending them accordingly is highly relevant. Fraunhofer FOKUS wants to elaborate and provide new insights for the industry. This is our ambition as one of the technological leaders in the European Open Data movement.
Q: What is the novelty of XMANAI and the main benefits envisaged for your organization?
A: The most exciting aspect of XMANAI from our perspective is data management. The data must be collected and prepared for the explainable AI models. Data pre-processing places completely different demands on data management than, for example, a traditional ETL process – since there are several user perspectives.
In industrial manufacturing, the individual machines and systems work reliably with each other like in a complex clockwork. Early detection of errors or necessary maintenance could prevent a system failure. Complex supply and production chains could also be optimised through pattern recognition. For this reason, data quality plays a significant role in developing AI models because the models can only be as good as the provided data.
An exciting aspect is to review existing metadata standards and data formats to see if they are still suitable for a scenario such as XMANAI. In the project context, it is also important to establish an exchange option for explainable AI models; here, additional provenance information must be collected and managed. Overall, there is a need for data management that is specially adapted to the XMANAI platform, and we see a lot of potential here.
Q: Which target groups can benefit from XMANAI?
A: Data engineers can benefit from XMANAI because the use of technologies for explainability has not yet been extensively studied. The entire data management process and life cycle of data and models should be (semi-)automated. This is challenging at the current stage and requires a special pre-processing (pipelines, etc.) of the data and metadata constructing a “glass box”.
For data scientists, the aspect of explainability of AI algorithms is undoubtedly interesting. Data schemes and structures need to be reviewed and possibly redesigned regarding their applicability. New approaches to the application of knowledge graphs are one possible outcome. New explainable AI models result from the XMANAI project can also be further explored and used.
Business users benefit through a better understanding of the application of artificial intelligence. The goal here is to generate trust so that decision-makers also increasingly use proven algorithms in the company or factory.
Q: How do you envision the XMANAI progress beyond the state of the art?
A: Research projects in the past have shown that the application of artificial intelligence or machine learning, in general, can have an advantage for a wide variety of business areas. However, for an all-encompassing deployment – i.e. the use of AI algorithms in critical systems – it is essential to understand how such an algorithm makes a decision.
The application of deep-learning and machine-learning algorithms and the extension of these is a challenge that must be addressed within XMANAI. For this purpose, the current technology must be analysed and questioned. Additionally, the resulting and explainable AI models should be easy to use, so they must run on different platforms – whether it is a local machine or a cloud application.
The use of knowledge graphs in combination with deep learning and machine learning algorithms in the context of manufacturing data goes beyond the state of the art because adequate graph support of the ML / DL libraries is not given. One possible reason is certainly the great flexibility of graph structures.
In addition, methods have to be developed to find valuable data or make it valuable for model generation. Usable AI models are only as good as the underlying data. In this context, it is equally essential that data protection and data security are addressed. Ethical guidelines should always be kept in mind.
Overall, the individual steps and results should help current manufacturing companies make a successful transition from a classic production environment into the AI era.
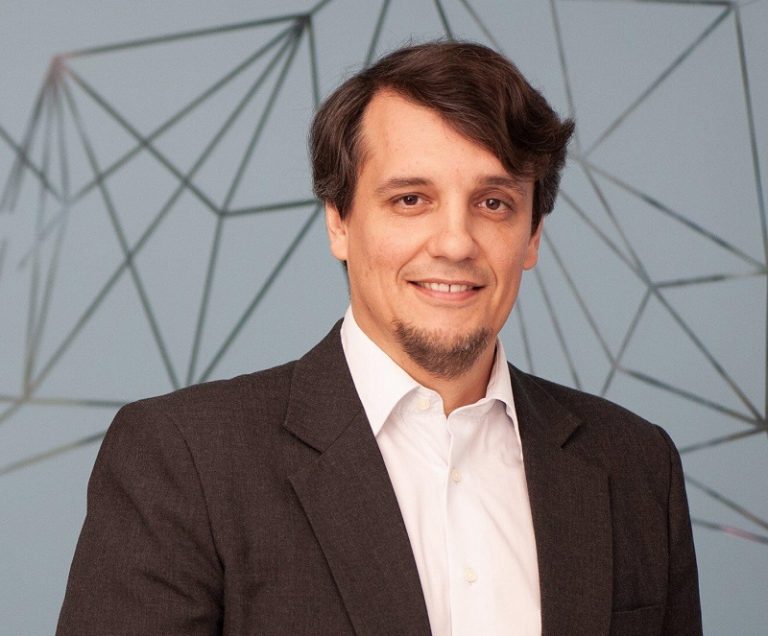
Organisation: Fraunhofer FOKUS
Dr. Yury Glikman is a researcher and a deputy-head of the Digital Public Services department at Fraunhofer FOKUS.
In the past 20 years, Yury was actively contributing and was leading technical activities in numerous international and national research projects. Yury is the coordinator of the H2020 projects ReachOut (The Beta Testing Campaign Platform for Research Projects), DataVaults (Persistent Personal Data Vaults Empowering A Secure And Privacy Preserving Data Storage, Analysis, Sharing And Monetisation Platform) and the scientific coordinator of the XMANAI project.
Yury is an elected member of the Directors Board Member of OW2 (www.ow2.org) – a global Open Source infrastructure software community. His current research interests focus on data integration, management and analytics.
Vincent Bohlen (male) Born in Berlin (Germany) in 1989. He received degrees in Computer Science at Freie Universität Berlin in 2014 (BSc) and 2017 (MSc).
He specialised in Networked-based Information Systems applying Artificial Intelligence methods to natural language data. After working as a Software Engineer NLP & AI, he joined Fraunhofer FOKUS as Researcher in 2018.
He is participating in various research projects concerning data infrastructures.
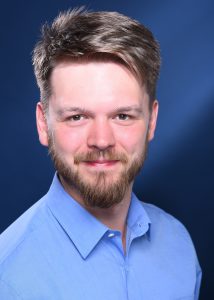
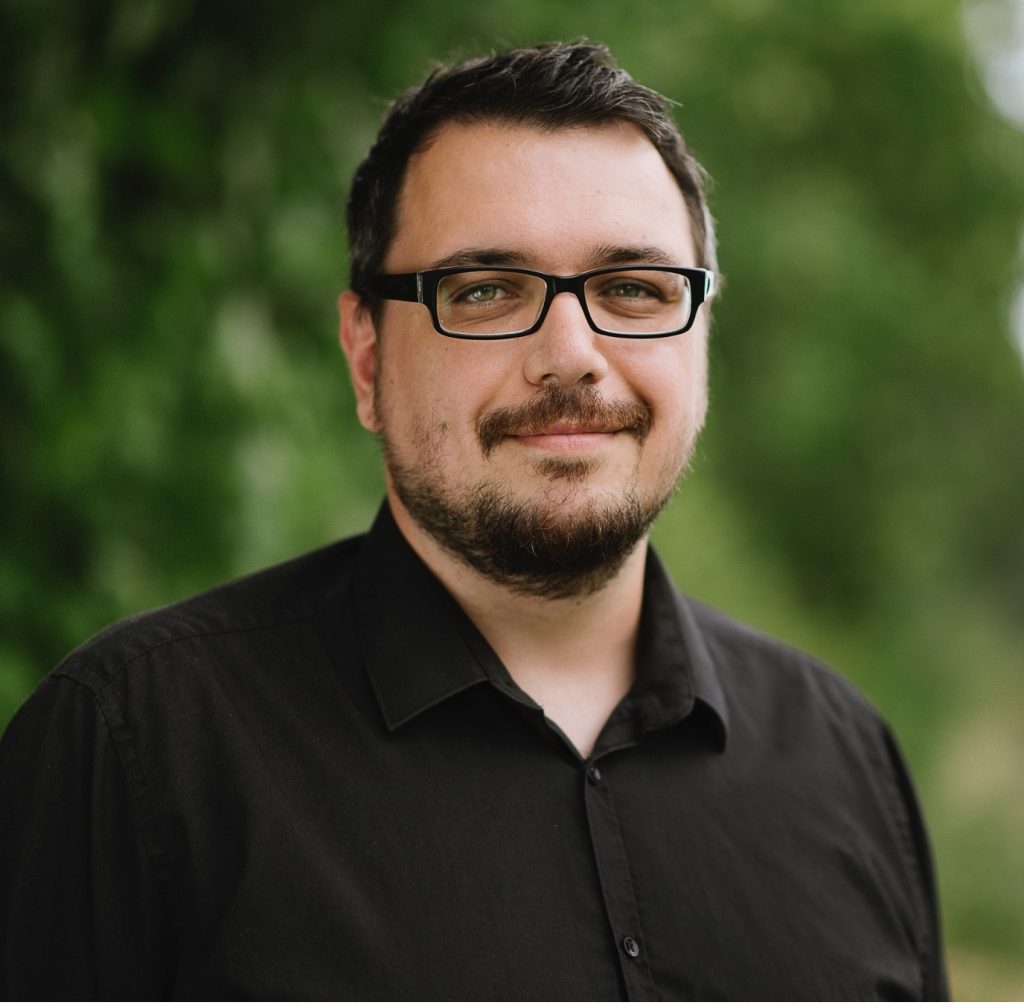
Sebastian Urbanek was born in Wesel (Germany) in 1985 and moved to Berlin in 2012. He studied Computer Science at Beuth University of Applied Sciences and completed his Master’s degree in 2017. In parallel to his studies, he worked at Carmeq GmbH (Volkswagen AG) and in the “MAGDa” research group at Beuth University later. Here, he focused on the analysis and visualisation of geodata using machine learning.
Since 2018, he started working at the Fraunhofer Institute for Open Communication Systems. He works as a researcher in the department “Digital Public Services”. The focus is on the topic of data management for open data.
He mainly works on the translation of datasets into all European languages. Furthermore, he develops statistics and analyses on the open data provided.