zExplAIn, Improving Manufacturing Processes with Explainable AI
Project Details and Motivation
In the new era of Industry 4.0, AI systems are becoming increasingly prevalent and cost-effective. With the ability to analyze vast amounts of data, factories can reduce expenses, boost productivity, and minimize waste. Overall, the potential of AI to solve previously intractable problems and improve efficiency is vast and continues to expand as new breakthroughs are made in the field.
One of the main issues with AI is that it can sometimes make decisions that are difficult for humans to understand. This lack of transparency and interpretability can make it challenging for humans to trust and use AI systems effectively. Additionally, they can sometimes exhibit bias or errors that are not immediately apparent, leading to inaccurate or unfair decisions.
Explainable AI (XAI) is a field that focuses on providing understandable explanations for AI system predictions and decision-making, which can improve trust, identify biases and errors, and lead to more accurate and fair decision-making. This growing field can aid manufacturing processes by making them more efficient and aware of the machines and products used, and at the same time, help managers make better decisions with expert algorithms that provide explanations for their predictions.
In Tyris AI, the implementation of this tool as a big part of our workflow has given us some insights both in the capabilities of this technology, and the promises that it is accomplishing.
For one, the explanations can help users find errors in the AI prediction systems when faced with unexpected situations or shifting environments with new data that the algorithm was not prepared to deal with. This not only makes the AI more reliable but also enables it to be tested to its limits, possibly extending the reach of the algorithm.
This not only improves the reliability and understanding of AI systems, but it also benefits in several other ways. XAI allows experts to identify biases that may be unintentionally built into AI models, ensuring fair and unbiased decision-making. Experts can also find errors in the AI system’s logic or assumptions, refining the model to improve its accuracy and reliability, which in turn enables it to help in more broad and critical scenarios.
XAI also enables developers to identify opportunities for expanding the tools and creating new products or services. With a better understanding of the AI system’s decision-making, it can be used to identify areas where the model can be further developed or applied, or even help in finding new sources of useful data for the problem by examining the explanations provided by the AI model, leading to improved accuracy and reliability of the predictions.
While working with Explainable AI algorithms, we discovered one of the main drawbacks of the current state of the art of this technology:
They are designed to provide explanations about the insights of the AI model, these explanations can often be overwhelming or too technical for non-expert users. This can render the explanations useless or too time-consuming to be practical. To address this issue, it is necessary to create a user interface that presents the explanations in a clear and user-friendly way. The interface should be designed to make it easy for non-technical users to understand the AI model’s decision-making process without needing to delve into the technical details of the algorithm.
In Tyris we have been working for the last two years on researching the topic of explainability in the XMANAI European Project. This has given us the expertise to develop our own approaches. Specifically, we had the opportunity to contribute to the Zero Defect Manufacturing Platform (ZDMP) project to add an XAI layer to provide insights to any industry AI project, and specifically to solve a Predictive Maintenance problem within the Engine Plant of the Ford Factory in Valencia, Spain.
Actually, we have addressed this challenge by creating a user oriented explainability layer that takes the technical explanations provided by the 3 different XAI algorithms and adapts them to the user’s environment to make it both more understandable and easier to visualize. As an example, we can provide a final analysis of the explanations to map the data to each component to a heatmap.
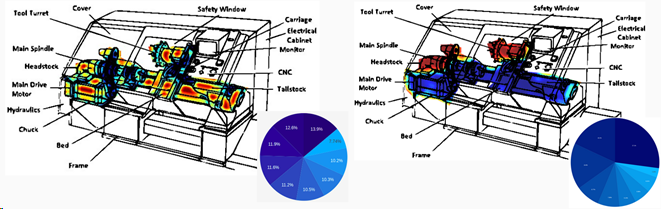
Our experience precedes us, as our project has been implemented in an offline on-premise environment, with all the challenges it implies. To achieve this, we created this as a self-contained application inside a containerized instance, with all the necessary components included. This entailed reliability and repeatability, but it also implied that Ford could easily move the project safely, with minimal of our assistance needed, and ensured the proper operation of the application in any condition or environment.
When integrated within the AI platform of the ZDMP project, everything was added to a pre-existing Kubernetes, which also required integration.
Results to Date
Although there were big topics to tackle along the way, new boundaries were to be explored.
The system was successfully integrated, expanding the capabilities of the pre-existing project, with all the requirements ready for the Ford Valencia Engine Plant. It started explaining the results that the models provided with a visually cozy interface.
Three existing explainability models were added to the project, allowing users to choose the one they are more comfortable with or the one that better fits their needs.
The deployment was positive, everything was prepared to be portable and easy to install.
The system was tested to work with the specifics of the environment where the project was deployed and validated.
Conclusions
In conclusion, the successful application of Tyris AI’s Explainable AI in ZDMP has once again demonstrated the immense potential of this technology to revolutionise the manufacturing industry. By providing clear and understandable explanations for the AI system’s decisions, we have created a tool capable of helping improve the efficiency of many critical and decision-making processes among many layers of the manufacturing process based on the insights provided by the algorithm.
Our approach has also shown the ability to apply XAI in challenging environments such as on-premise without an internet connection, paving the way for wider adoption of this technology across diverse industries.
We are proud of our accomplishments and excited to continue exploring the possibilities of XAI in the future.