Hackathon Event: Paving the Way to Transparent AI in Manufacturing with XMANAI
The XMANAI Hackathon, held in Athens, Greece on the 13th and 14th of July, provided a unique platform for students, data scientists, and industry experts to explore the critical need for explainability in AI applied to manufacturing. Organized by the XMANAI project and hosted by ATHENA Research Center, this event aimed to unravel the enigma of AI by delving into cutting-edge techniques for developing, interpreting, and implementing explainable AI (XAI) models. The ultimate goal was to dive deep into the XMANAI environment, and test and use the provided toolkit to create more transparent and interpretable AI systems, fostering trust and accountability.
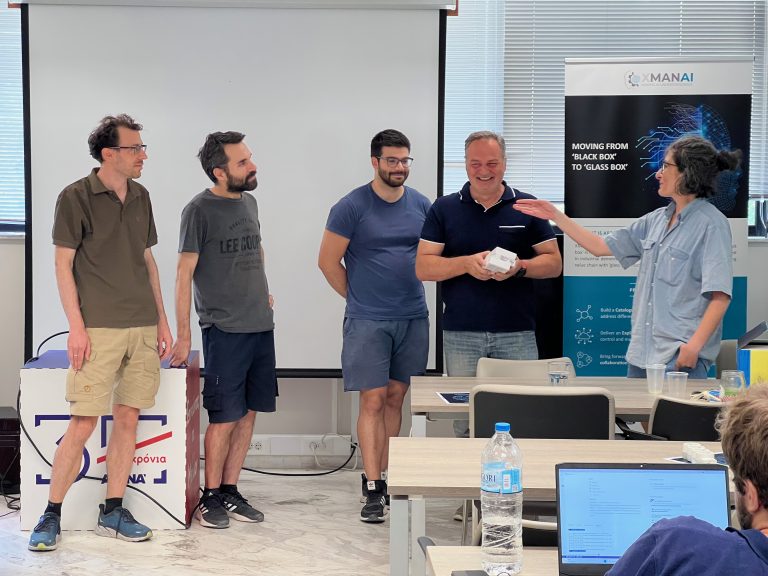
Understanding Explainable Artificial Intelligence (XAI)
The concept of Explainable AI (XAI) has gained momentum as a crucial field dedicated to making AI algorithms transparent and interpretable. Conventional AI models often function as “black boxes,” making it challenging for humans to comprehend the rationale behind their decisions. This lack of transparency raises concerns about biases, fairness, and accountability, particularly in scenarios with significant impacts on individuals and society.
XMANAI's Vision for Manufacturing
The XMANAI project focuses on manufacturing, striving to make AI models in this domain step-by-step understandable and actionable at various levels, from data exploration and processing to final results. By promoting transparency and interpretability in AI for manufacturing, XMANAI aims to empower users with a deeper understanding of AI systems’ decision-making processes.
The Hackathon: A Competition with Real-World Data
The event provided hands-on experience, tackling real-world problems on machinery control and industrial metrology related to XMANAI industrial pilots and applying the existing XAI framework to analyze real data. Supervised hands-on activities in the context of the XMANAI industrial pilots were performed on the XMANAI platform, showcasing its experimentation functionalities.
Covering yet another aspect of XAI, a tutorial and hands-on session dedicated to assessing the fairness of AI models towards sensitive societal groups with use of XAI methods was guided by experts participating in the Autofair project. Participants in the hackathon were therefore exposed to different real-world scenarios and the corresponding explainability needs to be met in each case.
Challenges and Learning
Over two days, participants in the XMANAI Hackathon formed teams of three members, collaborating to develop explainable AI solutions. The participants faced numerous challenges during the Hackathon, including working with complex problems and explainable AI models.
The event encouraged creative problem-solving and critical thinking, enabling attendees to enhance the transparency and interpretability of AI systems. By combining theory with practical application, the Hackathon offered valuable insights into the world of XAI and how it can be used to drive advancements in manufacturing.
Hackathon Results
The XMANAI AI Explainability Hackathon garnered significant visibility, attracting 21 participants, with 15 actively engaged in the competition, organized into five groups. Very interesting XAI solutions were presented by all competing teams, making the evaluation of the projects a truly difficult task. The projects finally winning the competition were those focusing on the provision of different types of explanations, synthesized in a complementary manner to explain multiple aspects of the respective AI solutions, rather than those focused on enhancing AI model performance.
The first award was handed to team Yoshimi which stood out in this respect. They:
- tackled the problem of point measurement error prediction, in the context of industrial metrology with the use of a 3D laser scanner;
- re-structured the supervised regression task of point measurement error prediction presented in the tutorials into a supervised binary classification setting, to discriminate between good (label=0) and bad (label=1) measurements, based on a certain threshold;
- employed a Random Forest ensemble to solve the classification task, and utilized three (3) post-hoc XAI techniques to explain the most important features affecting the ‘black-box” model’s predictions: LIME, SHAP, and Counterfactual Analysis. Interestingly, counterfactual explanations were focused into important parameters of the scanning setup that can be configured by the operator in order to change a bad prediction into a good one (Incidence Angle of laser light on the surface, Viewing Angle by the camera sensor);
- also applied an Explainable Boosting Machine (EBM) to the task: this is a high-performing, interpretable by-design model that also considers input feature interactions.
The quality of the proposed solutions is impressive and shows that participants were genuinely engaged to understand the problems put forth by the manufacturing pilots and, more importantly, to address the need for interpretable solutions and explanations in each case.
The event also generated a strong social media presence, with at least 38 tweets, 78 reactions, and 2849 views on Twitter. On LinkedIn, eight posts reached a broader audience, sparking 106 reactions and 2503 impressions.
In conclusion, the XMANAI AI Explainability Hackathon was a pivotal event, validating the XAMANAI framework, and advancing the understanding and implementation of explainable AI in manufacturing. The event was able to promote collaboration, problem-solving, and innovation by bringing together a diverse group of participants.
The focus on XAI empowered individuals to comprehend AI decisions, promoting responsible and ethical AI applications. As we embrace an AI-driven future, the XMANAI project’s efforts play a vital role in shaping AI’s evolution, making it transparent, interpretable, and trustworthy. By fostering a community dedicated to ethical AI advancements, XMANAI is positively impacting manufacturing and beyond.