Models Catalog Component of the Platform
In the dynamic realm of Artificial Intelligence (AI) applied to manufacturing, XMANAI (eXplainable Manufacturing Artificial Intelligence) emerges as a platform designed to cater to professionals seeking not only advanced AI capabilities but also a profound understanding of their models. At the heart of this transformative platform lies the XMANAI Models Catalog, a robust repository meticulously crafted to store and link data, models, and explainers seamlessly.
A pivotal component ensuring a smooth user experience, the Models Catalog plays a crucial role every time a model is trained. The transparently integrated Execution and Orchestration Engine effortlessly calls upon the catalog, establishing vital linkages that enhance the overall efficiency of the AI training process and ecosystem.
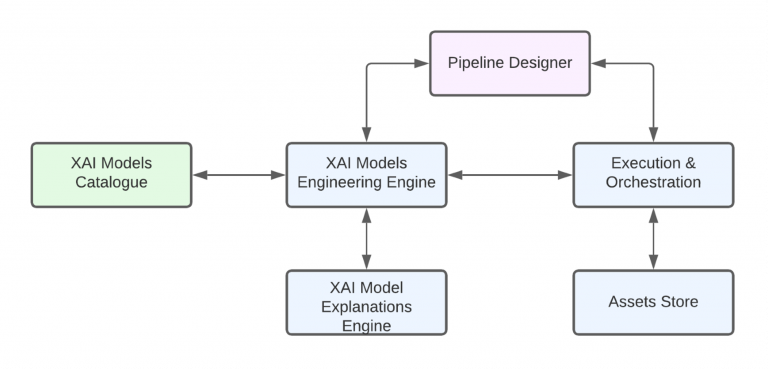
Structured Data for Seamless Treatment:
For optimal functionality, training files uploaded through the Models Catalog must adhere to a specific structure. This meticulous approach guarantees that data is treated with precision, paving the way for a seamless integration of our various model types, and ensuring the important explainability component of the platform.
This is especially important since from the beginning we set as our design requirements allowing the platform to train many kinds of models, but specially Hybrid Models and cutting-edge Graph Models.
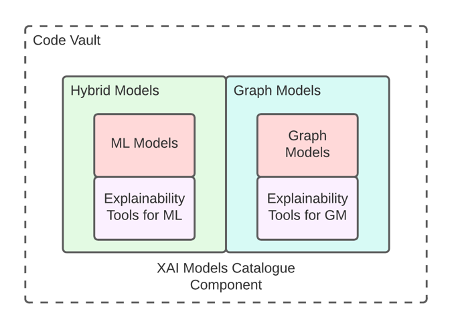
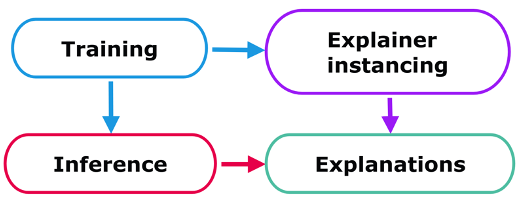
Hybrid Models: Bridging the Gap to Explainability
XMANAI stands out by supporting both hybrid (ML + Explainer) and Graph Machine Learning models. The design philosophy behind hybrid models is particularly noteworthy, as it ensures explainability regardless of the intrinsic interpretability of the model itself. Even in cases where the model lacks inherent explainability, a model-agnostic explainer is readily available to shed light on the decision-making process.
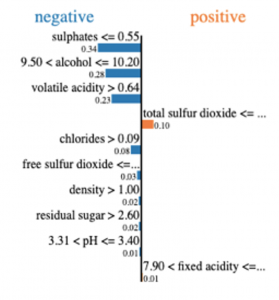
Graph Models: Navigating Complexity with Simplicity
The vangard Graph Machine Learning models, a highlight of the XMANAI platform, excel in solving intricate manufacturing problems. Leveraging attention layers, these models are inherently explainable, simplifying the transmission of human expert knowledge into the AI system.
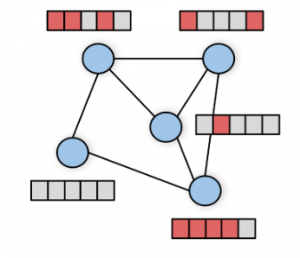
Real Solutions for Real Problems
The development team behind XMANAI has used the platform to address four real manufacturing challenges in their demonstrators (Ford, Whirlpool, Unimetrik, CNH). From anomaly detection in manufacturing processes to optimizing process scheduling, the platform has proven instrumental in delivering effective solutions. The success of these real-world applications underscores the practicality and effectiveness of the XMANAI platform. Each of the demonstrators presented unique hurdles, and the XMANAI platform, in tandem with the Models Catalog, adapted to provide tailored solutions.
Explainability as a Cornerstone
A defining feature of XMANAI is its commitment to explainability, which is upheld by the indispensable role of the Models Catalog.
This catalog ensures that decisions and predictions made by the models are transparent, allowing technical partners to comprehend biases and errors during the development phase. The Models Catalog acts a vital role facilitating the seamless integration of explainers, which not only instills confidence in end-users but also facilitates trust in critical processes where the models may play a pivotal role. This enforcement of transparency is a testament to the meticulous design and functionality of the Models Catalog, making it an integral part of XMANAI’s success in delivering explainable AI solutions.
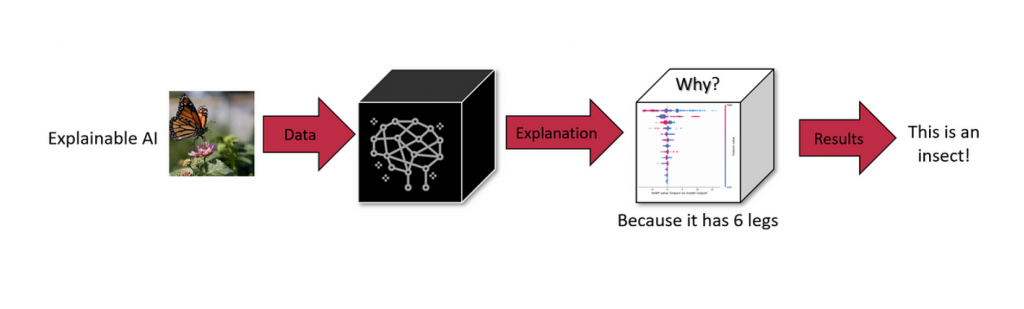