Knowledge Transfer and Replication Roadmap
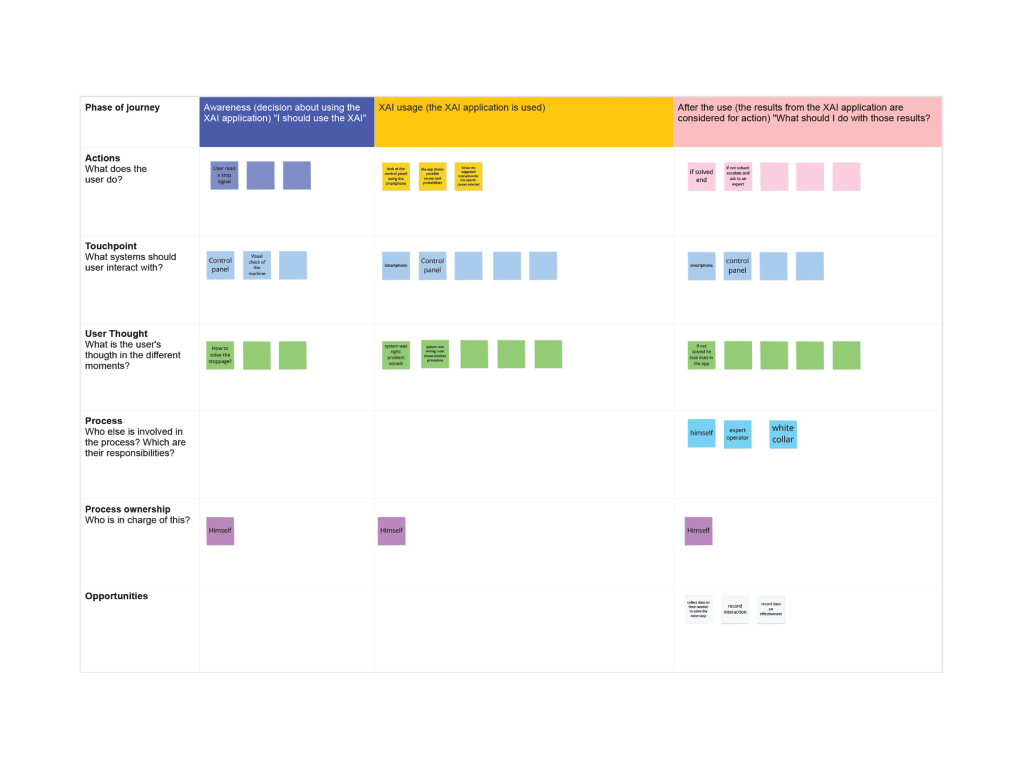
Knowledge Transfer and Replication Roadmap The present post provides recommendations and guidelines for porting scaling up and replication the XMANAI concept at larger scales and for transferring knowledge of XMANAI and the developed assets and infrastructure to other manufacturing sectors and domains. It offers a structured framework to guide an organization’s thought processes during the […]
XMANAI Scientific Highlights and Publications
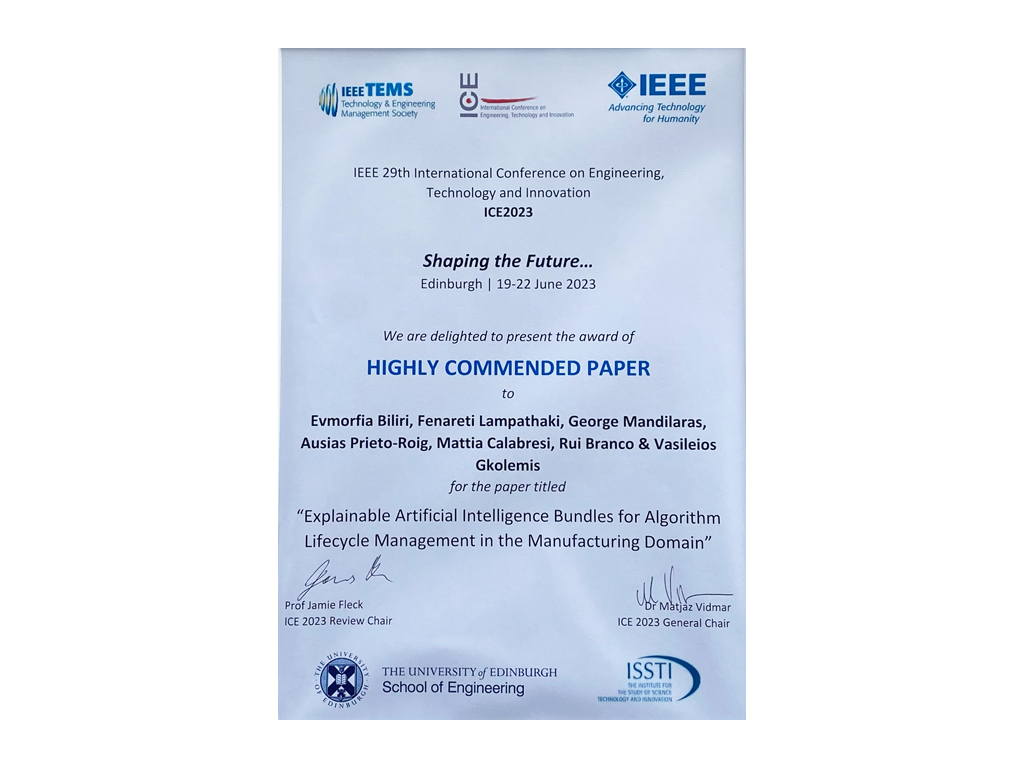
XMANAI Scientific Highlights and Publications XMANAI has made significant strides in its outreach and dissemination efforts, producing several scientific publications that showcase its advancements in explainable AI for manufacturing. We are thrilled to announce the successful publication of 21 research papers, with 12 presented at peer-reviewed conferences and 3 in esteemed journals, each contributing valuable […]
X-by-Design
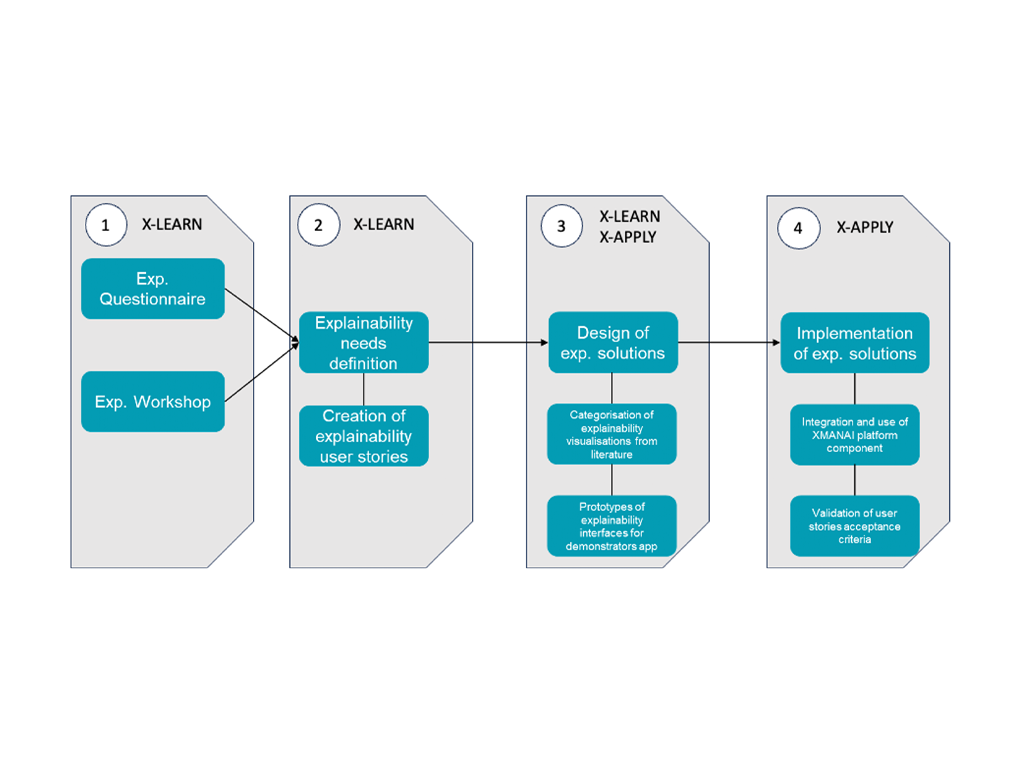
X-by-Design Integrating Explainability-by-Design for Transparent and Efficient AI in Manufacturing Artificial intelligence (AI) is revolutionizing industries, but its complexity often makes it opaque and hard to interpret, and in some cases not accepted by end-users. This opacity has led to the rise of Explainable AI (XAI), which aims to make AI systems’ decisions understandable to […]
XAI for Metrology at UNIMETRIK: Smart Semi-autonomous Hybrid Measurement Planning

XAI for Metrology at UNIMETRIK: Smart Semi-autonomous Hybrid Measurement Planning Optimal parameters use case The UNIMETRIK demonstrator use Explainable AI to reduce the time invested in the measuring process and to increase the accuracy of the measurements performed with M3. A double benefit is achieved: • The XMANAI platform contribute to “reuse” relevant information such as key […]
Towards Process and Production Optimisation with XAI:The CNH Use Cases

Towards Process and Production Optimisation with XAI: The CNH Use Cases CNH Industrial is a world-class equipment and services company, a global leader in the design and manufacturing of agricultural and construction machines, that employs more than 64.000 people in 66 manufacturing plants and 54 R&D centers in 180 countries. The collaboration with European partners […]
Holistic Production Overview using XAI technologies: The Ford use cases

Holistic Production Overview using XAI technologies: The FORD use cases Ford Motor Company is a global automotive and mobility company. The Company’s business includes designing, manufacturing, marketing, and servicing a full line of Ford cars, trucks, and sport utility vehicles. Ford plants and offices are located in every region of the world, employing in 2020, […]
XAI Applications in the Direct to Consumer (D2C) market. The Whirlpool use cases
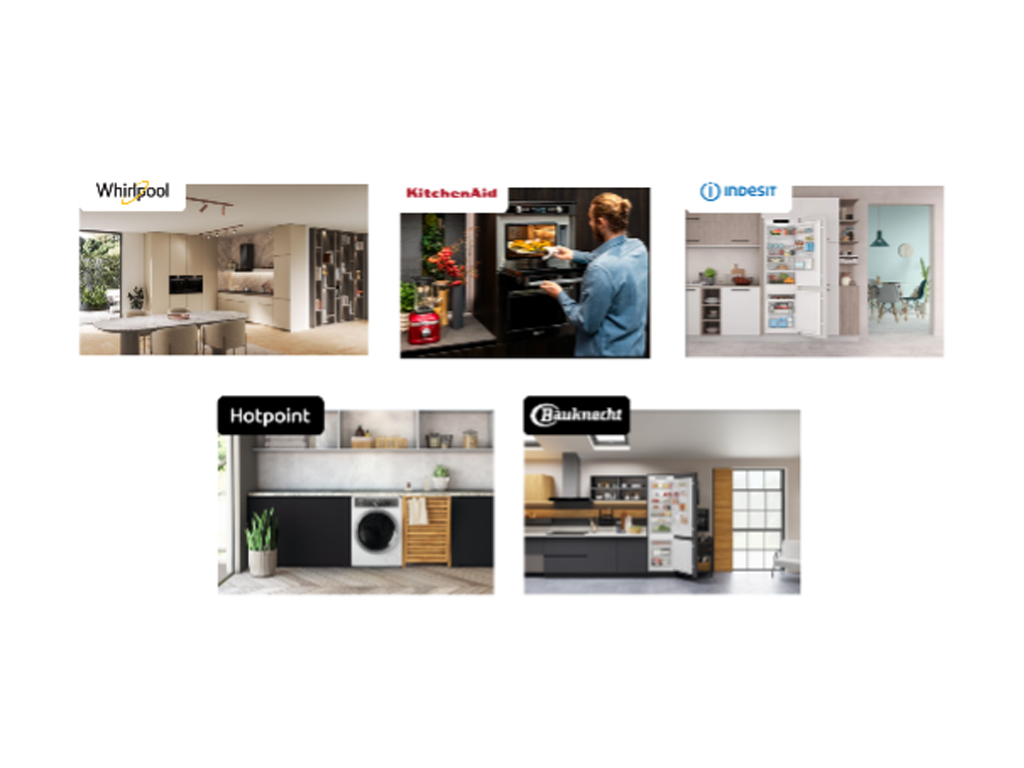
XAI Applications in the Direct to Consumer (D2C) market. The Whirlpool use cases Whirlpool Corporation is the world’s leading kitchen and laundry appliance company, with approximately $19 billion in annual sales, 78,000 employees, and 57 manufacturing and technology research centers in 2020. With a sales presence in more than 35 countries and manufacturing sites in […]
XMANAI Provenance Engine: Tracking data provenance and data lineage
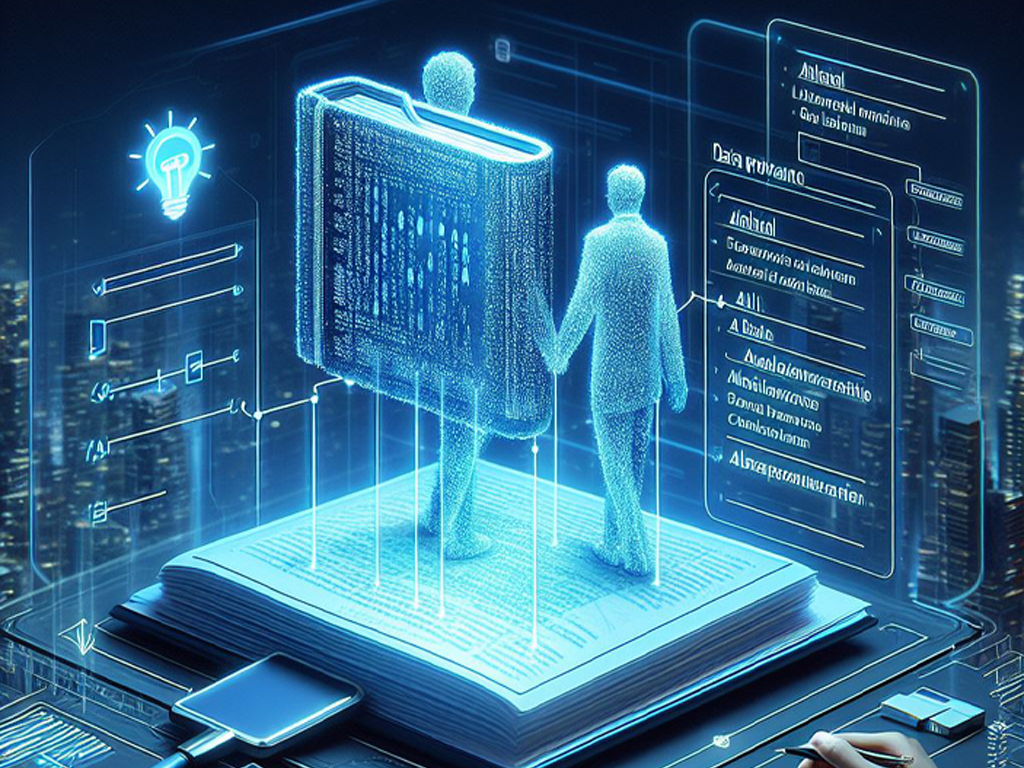
XMANAI Provenance Engine: Tracking data provenance and data lineage What is Data Provenance and Why is it Important? Data provenance is akin to a comprehensive breadcrumb trail for data, tracing a dataset from its origins to consumption, and any transformations along the way. More specifically, data provenance records are metadata that tracks what changes are […]
The XMANAI Platform and Tools Package
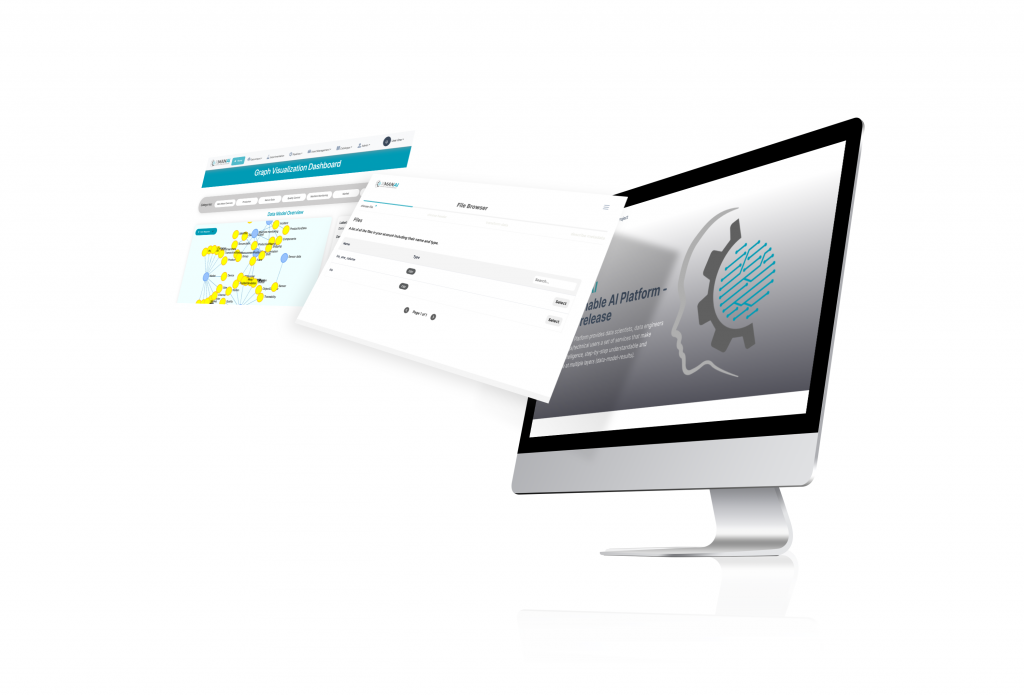
The XMANAI platform and Tools Package After the successful launch of the Beta Version, the XMANAI team is thrilled to announce the release of the much-anticipated Final Version of the XMANAI Platform. The last iteration marks a significant milestone in our journey toward revolutionizing the way AI and XAI (Explainable AI) are integrated and utilized […]
XMANAI On Premise Environment
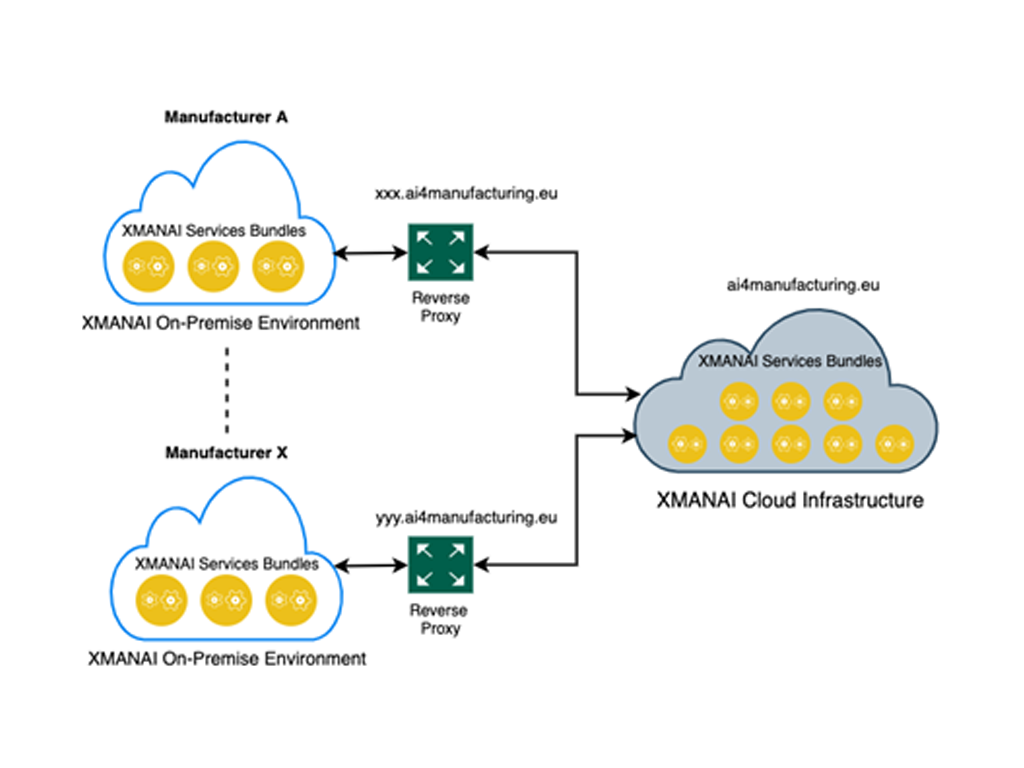
XMANAI On-premise execution and visualization environment: A Deep Dive into the Final Release Version The XMANAI platform offers a cutting-edge approach to Explainable Manufacturing Artificial Intelligence and has recently unveiled its Beta Version, building upon the solid foundation laid by the Alpha release. As has been presented in the past, the XMANAI Platform architecture comprises […]
Models Catalog Component of the Platform
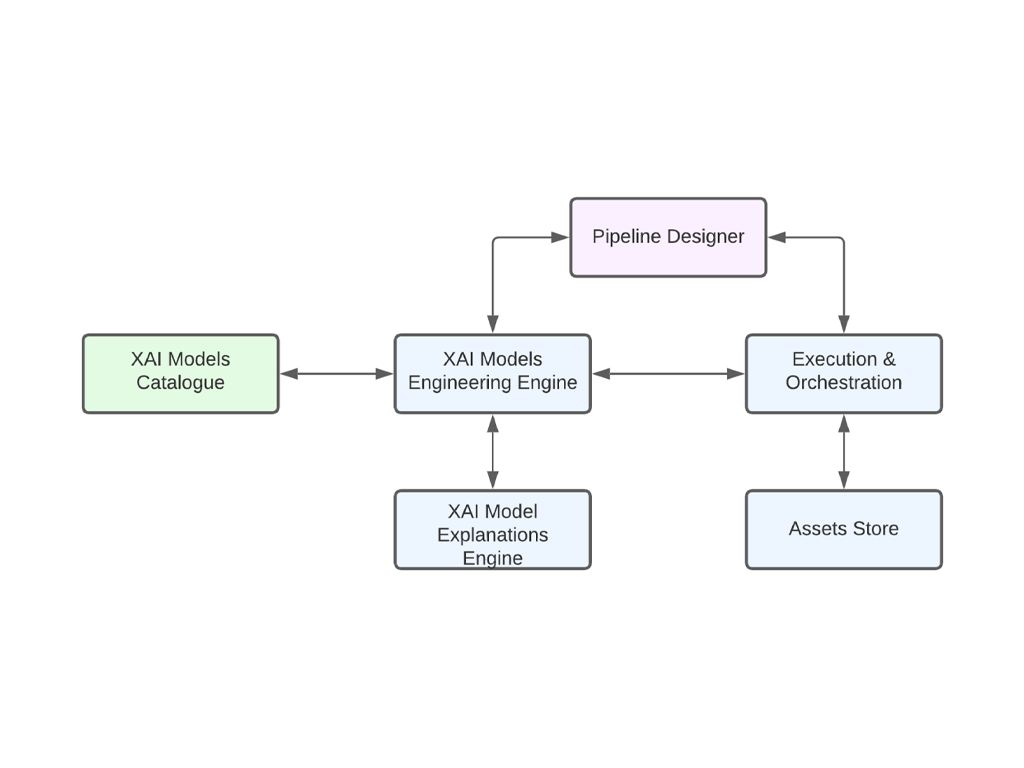
Models Catalog Component of the Platform In the dynamic realm of Artificial Intelligence (AI) applied to manufacturing, XMANAI (eXplainable Manufacturing Artificial Intelligence) emerges as a platform designed to cater to professionals seeking not only advanced AI capabilities but also a profound understanding of their models. At the heart of this transformative platform lies the XMANAI […]
Explaining Transformers
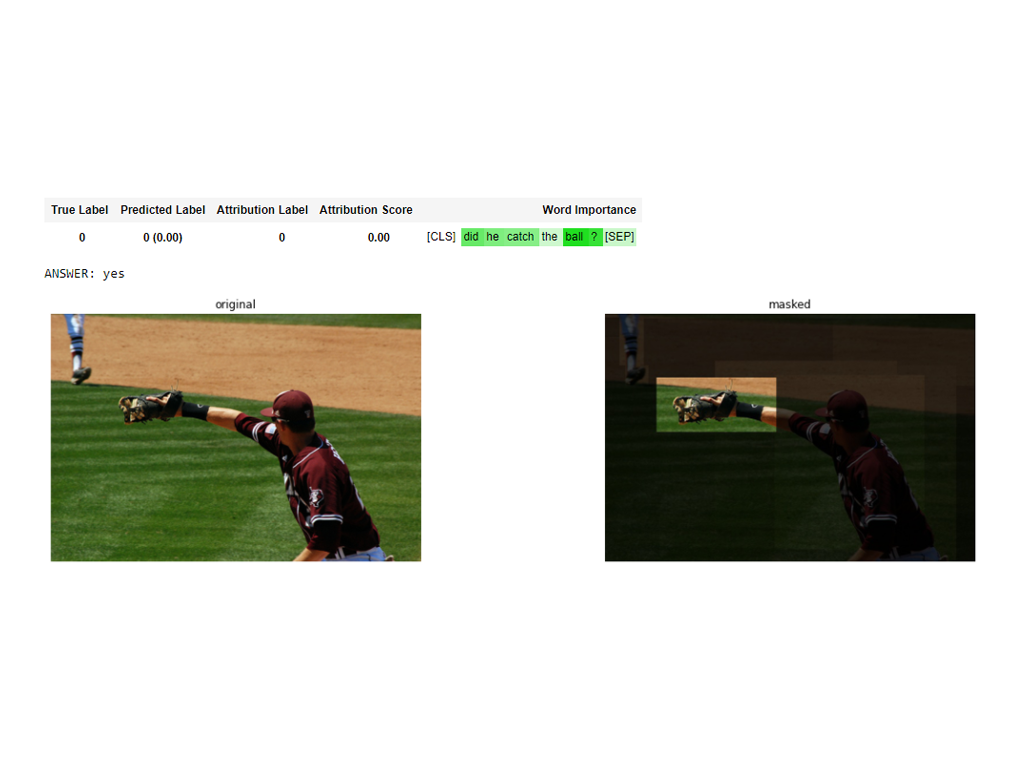
Explaining Transformers Transformers are neural network architectures that have delivered performant solutions in several fields including Natural Language Processing (NLP), computer vision & audio/speech analysis. In fact, state-of-the-art NLP models such as GPT4 and BERT are built using transformer blocks. The self-attention mechanisms upon which these models are built allow for parallel processing of the […]
How are the final MVP features contributing to the X-By-Design Concept?
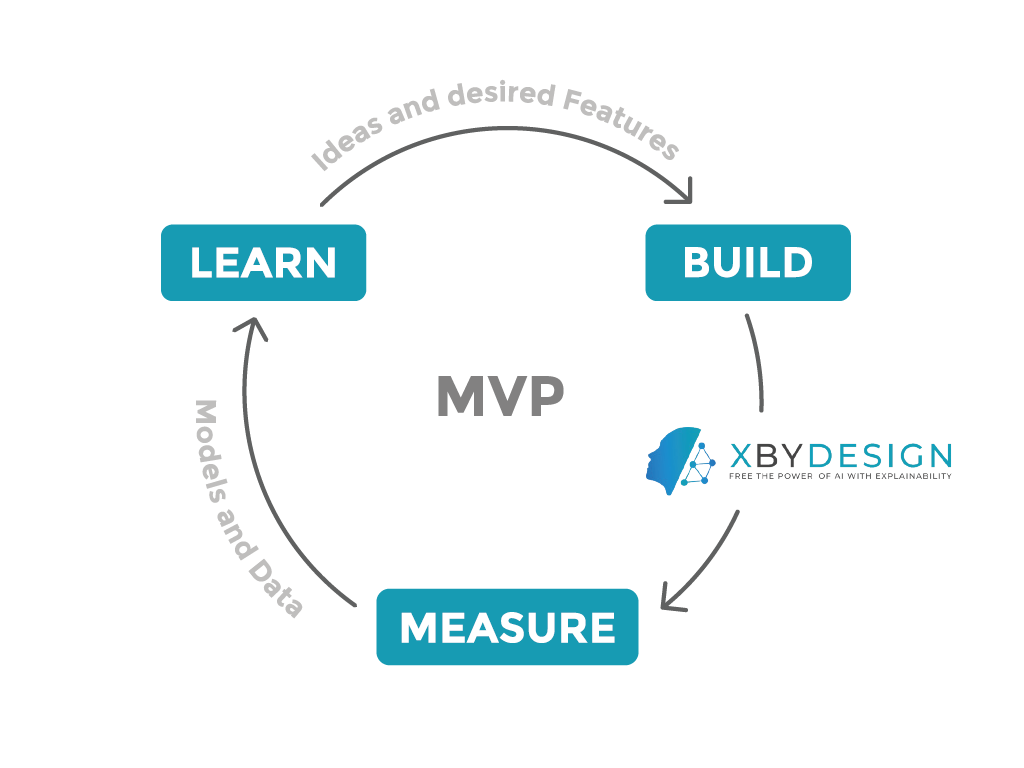
How are the final MVP features contributing to the X-By-Design Concept? Introduction to the Final MVP As already discussed in a previous post, the XMANAI Minimum Viable Product (MVP) is designed with the minimum set of features and functionalities that can satisfy early adopters who, in turn, can promptly provide feedback for future improvements. During […]
The Power of X-by-design: Pioneering Transparency and Explainability through Design
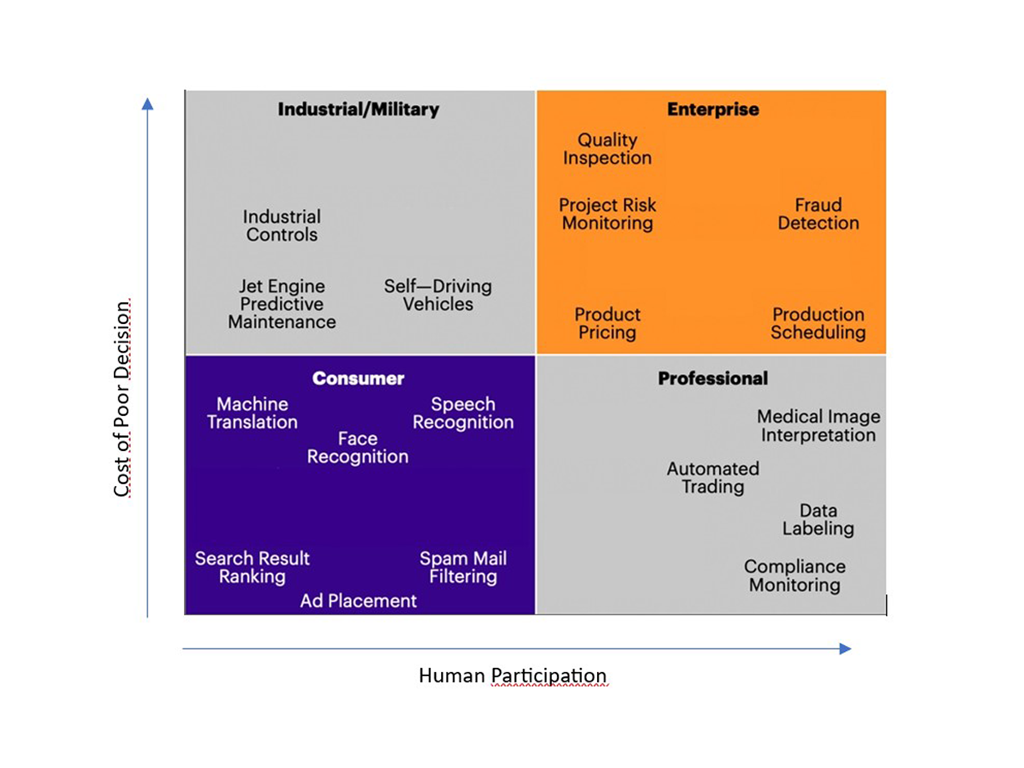
The Power of X-by-design: Pioneering Transparency and Explainability through Design In recent years, artificial intelligence (AI) has rapidly transformed various industries, revolutionizing how we interact with technology and process information. However, alongside these advancements, concerns about the transparency and interpretability of AI systems have emerged. The concept of “explainable AI” (XAI) has thus gained significant […]
Hackathon Event: Paving the Way to Transparent AI in Manufacturing with XMANAI
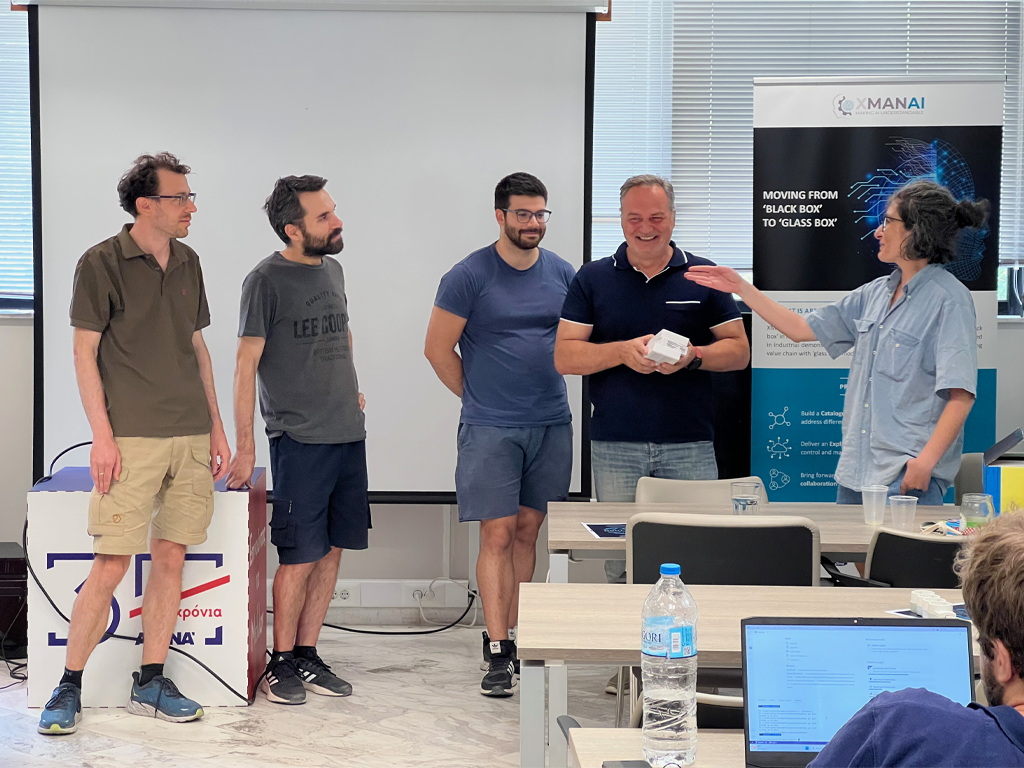
The XMANAI Hackathon, held in Athens, Greece on the 13th and 14th of July, provided a unique platform for students, data scientists, and industry experts to explore the critical need for explainability in AI applied to manufacturing.